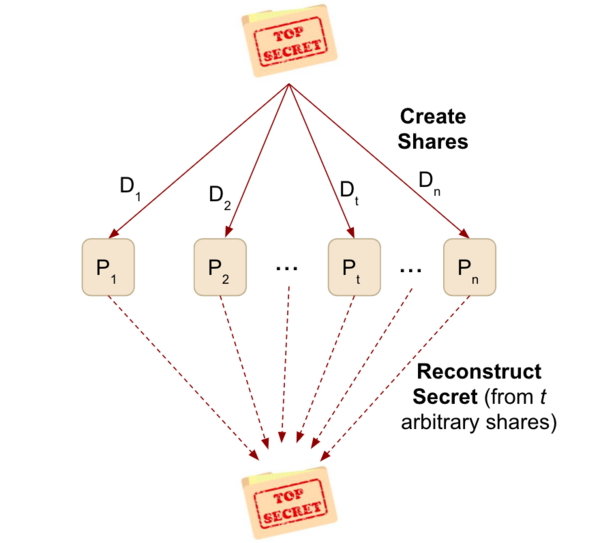
In this study, the authors develop an architecture to implement in a cloud-based database used by law firms to ensure confidentiality, availability, and integrity of attorney documents while maintaining greater efficiency than traditional encryption algorithms. They assessed whether the architecture satisfies necessary criteria and tested the overall file sizes the architecture could process. The authors found that their system was able to handle larger file sizes and fit engineering criteria. This study presents a valuable new tool that can be used to ensure law firms have adequate security as they shift to using cloud-based storage systems for their files.
Read More...