Prediction of preclinical Aβ deposit in Alzheimer’s disease mice using EEG and machine learning
(1) University High School, Irvine, California, (2) Department of Anatomy and Neurobiology, University of California, Irvine, California
https://doi.org/10.59720/22-110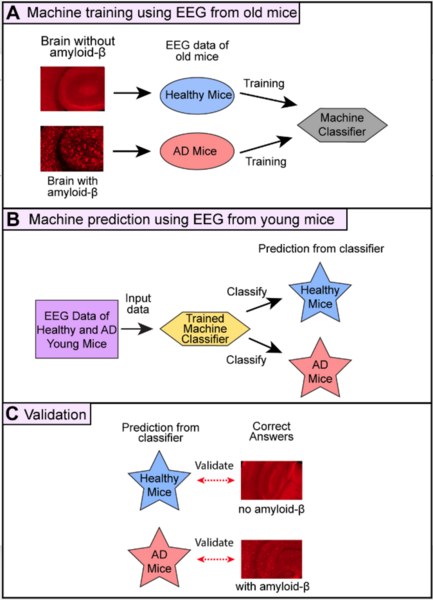
Alzheimer’s disease (AD) is a common disease affecting 6 million people in the U.S., but no cure exists. To create therapy for AD, it is critical to detect amyloid-β protein in the brain at the early stage of AD because the accumulation of amyloid-β over 20 years is believed to cause memory impairment. However, it is difficult to examine amyloid-β in patients’ brains. The development of a simple method to predict the presence of amyloid-β without harming patients would be helpful for clinical applications. Electroencephalography (EEG) is a testing method that records brain activity using electrodes attached to the scalp without harming the brain. In this study, we hypothesized that we could accurately predict the presence of amyloid-β using EEG data and machine learning. To test this, we analyzed published EEG data recorded from healthy mice and AD mice. Machine classifiers were trained using EEGs of old mice with and without amyloid-β. We then tested if the best trained machine classifier (Gaussian Naïve Bayes (GNB) classifier) could predict amyloid-β in young mice from their EEG data. Results showed that GNB classifier can predict if a given mouse has amyloid-β or not at 81% accuracy when theta waves were used for classifier training. The accuracy of the classifier was at chance level when beta or gamma waves were used. These results indicate that amyloid-β presence can be predicted at high accuracy using EEG and machine learning and suggest that this method may be useful for early diagnosis of AD patients.
This article has been tagged with: