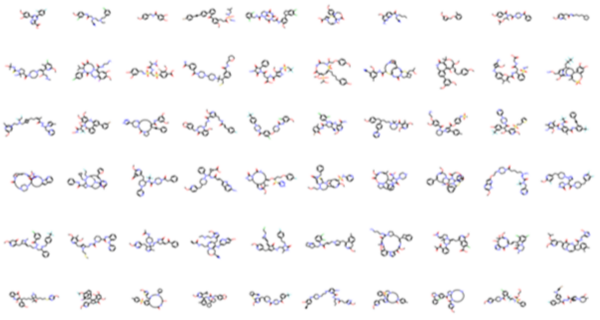
The authors investigate the ability of machine learning models to developing new drug-like molecules by learning desired chemical properties versus simply generating molecules that similar to those in the training set.
Read More...Evaluating the feasibility of SMILES-based autoencoders for drug discovery
The authors investigate the ability of machine learning models to developing new drug-like molecules by learning desired chemical properties versus simply generating molecules that similar to those in the training set.
Read More...Cytotoxicity evaluation of Amaranthus extracts compared with AS20 on MCF-7 cancer cells
The authors test the antiproliferative and apoptosis-inducing properties of an extract created from a traditional Indian medicinal plant of the Amaranthus genus.
Read More...Optimizing airfoil shape for small, low speed, unmanned gliders: A homemade investigation
Here, the authors sought to identify a method to optimize the lift generated by an airfoil based solely on its shape. By beginning with a Bernoullian model to predict an optimized wing shape, the authors then tested their model against other possible shapes by constructing them from Styrofoam and testing them in a small wind tunnel. Contrary to their hypothesis, they found their expected optimal airfoil shape did not result in the greatest lift generation. They attributed this to a variety of confounding variables and concluded that their results pointed to a correlation between airfoil shape and lift generation.
Read More...Testing HCN1 channel dysregulation in the prefrontal cortex using a novel piezoelectric silk neuromodulator
Although no comprehensive characterization of schizophrenia exists, there is a general consensus that patients have electrical dysfunction in the prefrontal cortex. The authors designed a novel piezoelectric silk-based implant and optimized electrical output through the addition of conductive materials zinc oxide (ZnO) and aluminum nitride (AlN). With further research and compatibility studies, this implant could rectify electrical misfiring in the infralimbic prefrontal cortex.
Read More...Understanding the Mechanism of Star-Block Copolymers as Nanoreactors for Synthesis of Well-Defined Silver Nanoparticles
Here, the authors characterize how silver ions nucleate a star-block copolymer to generate nano-sized silver particles.
Read More...Recognition of animal body parts via supervised learning
The application of machine learning techniques has facilitated the automatic annotation of behavior in video sequences, offering a promising approach for ethological studies by reducing the manual effort required for annotating each video frame. Nevertheless, before solely relying on machine-generated annotations, it is essential to evaluate the accuracy of these annotations to ensure their reliability and applicability. While it is conventionally accepted that there cannot be a perfect annotation, the degree of error associated with machine-generated annotations should be commensurate with the error between different human annotators. We hypothesized that machine learning supervised with adequate human annotations would be able to accurately predict body parts from video sequences. Here, we conducted a comparative analysis of the quality of annotations generated by humans and machines for the body parts of sheep during treadmill walking. For human annotation, two annotators manually labeled six body parts of sheep in 300 frames. To generate machine annotations, we employed the state-of-the-art pose-estimating library, DeepLabCut, which was trained using the frames annotated by human annotators. As expected, the human annotations demonstrated high consistency between annotators. Notably, the machine learning algorithm also generated accurate predictions, with errors comparable to those between humans. We also observed that abnormal annotations with a high error could be revised by introducing Kalman Filtering, which interpolates the trajectory of body parts over the time series, enhancing robustness. Our results suggest that conventional transfer learning methods can generate behavior annotations as accurate as those made by humans, presenting great potential for further research.
Read More...Error mitigation of quantum teleportation on IBM quantum computers
Quantum computers can perform computational tasks beyond the capability of classical computers, such as simulating quantum systems in materials science and chemistry. Quantum teleportation is the transfer of quantum information across distances, relying on entangled states generated by quantum computing. We sought to mitigate the error of quantum teleportation which was simulated on IBM cloud quantum computers.
Read More...The effects of Helianthus Annuus on Amyotrophic Lateral Sclerosis using Drosophila Melanogaster
Amyotrophic lateral sclerosis (ALS) affects nearly 200,000 people worldwide and there is currently no cure. The purpose of the study was to determine if Helianthus annuus seeds helped reduce nerve degeneration and increase locomotion using Drosophila melanogaster as the model organism. Through this experiment, we found a general trend suggesting that H. annuus helped increase the mobility of the D. melanogaster suggesting it could be a viable supplement for patients with ALS.
Read More...Identifying Neural Networks that Implement a Simple Spatial Concept
Modern artificial neural networks have been remarkably successful in various applications, from speech recognition to computer vision. However, it remains less clear whether they can implement abstract concepts, which are essential to generalization and understanding. To address this problem, the authors investigated the above vs. below task, a simple concept-based task that honeybees can solve, using a conventional neural network. They found that networks achieved 100% test accuracy when a visual target was presented below a black bar, however only 50% test accuracy when a visual target was presented below a reference shape.
Read More...Utilizing the Magnus effect to produce more downforce than a standard wing
Here, seeking a better solution to produce downforce that keeps a vehicle grounded at high speeds than wings which tend to result in degraded car performance due to increased air resistance, the authors considered using the Magnus effect as a replacement. The authors found that a spinning cylinder generated significantly more downforce through the Magnus effect than a standard wing at all wind speeds as simulated through the use of a leaf blower. They suggest that a cylinder could be a potential replacement for a wing when downforce is a priority.
Read More...