Evaluating the feasibility of SMILES-based autoencoders for drug discovery
(1) Carlmont High School
https://doi.org/10.59720/22-248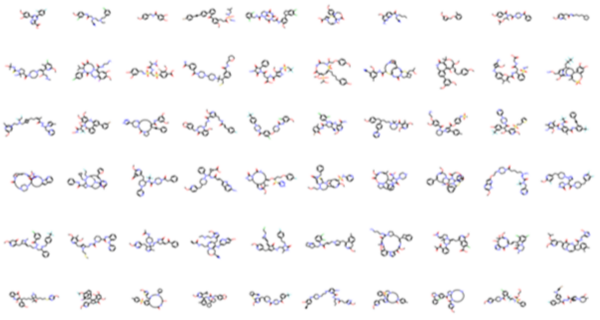
The vast majority of molecules with desirable drug-like properties have not yet been discovered. With the advent of machine learning for de novo molecular generation, the process of designing these molecules has become increasingly efficient. However, to what extent are these machine learning models actually learning chemical properties versus memorizing the syntax of a training set? In this project, we trained a Simplified Molecular Input Line Entry System (SMILES)-based generative autoencoder for up to 200 epochs to investigate whether the latent space can separate molecules based on five chemical properties (partition coefficient, molecular weight, topological polar surface area, number of hydrogen bond donors, and number of hydrogen bond acceptors) and how generated molecules compare to the training set. We hypothesized that the model would preferentially encode molecular weight and that generated molecules would be similar to the training set. Consistent with our hypothesis, the model quickly learned to distinguish molecules primarily by their molecular weight, while other properties were considered to a lesser extent. Moreover, generated molecules were very similar to the training set both in terms of structure and properties. These results suggested that the model overfits the training set. In particular, the model best learns chemical properties that directly depend on atomic composition while it is difficult for the model to encode higher-level properties that rely on connectivity and structure. Our results may represent fundamental limitations of SMILES-based generative models and could assist in development of new research to mitigate these issues.
This article has been tagged with: