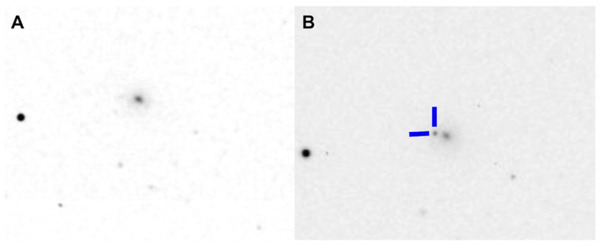
Observing transients like supernovae, which have short-lived brightness variations, helps astronomers understand cosmic phenomena. This study analyzed transient 2023jri, hypothesizing it was a Type IIb supernova. By collecting and analyzing data over four weeks, including light and color curves, they confirmed its classification and provided additional insights into this less-studied supernova type.
Read More...