Artificial intelligence assisted violin performance learning
(1) BASIS Oro Valley, Tucson, Arizona, (2) AIClub, Pyxeda AI, Saratoga, California
https://doi.org/10.59720/22-264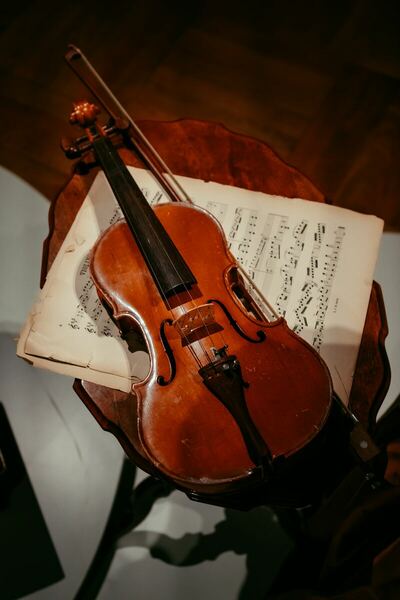
While learning the violin has many benefits, some people don't have access to teachers. Furthermore, music students typically have a week in between lessons, during which time they lack guidance – feedback that can greatly improve performance – on their practice. Our study explores an “artificial music tutor” for assisting violinists. We aimed at detecting major solfège errors in instrumental play. We hypothesized that artificial intelligence (AI) algorithms Random Forest, K Nearest Neighbor, and Multilayer Perceptron would identify errors. We further hypothesized that Random Forest would perform the best given its viability across applications requiring classification tasks. To build the AI, we gathered violin recordings played correctly and with errors, which we translated into a featurized dataset. We trained AI algorithms to distinguish between correct and incorrect recordings using two datasets. The first dataset contained one correct recording and three incorrect intonation, rhythm, and tempo recordings per piece. We achieved 100% accuracy with Random Forest in detecting recordings. The second dataset contained two correct recordings and three incorrect intonation, rhythm, and tempo recordings per piece. The highest accuracy was 71.42% for distinguishing between both incorrect tempo vs. intonation with Random Forest and incorrect rhythm vs. intonation with Multilayer Perceptron. Our findings support our hypothesis that Random Forest is generally the most accurate algorithm. However, Multilayer Perceptron also achieved the highest accuracy for the second dataset, so we concluded it is suitable for identifying performance errors. This AI will assist musicians in practicing without a teacher, showing players where they can improve.
This article has been tagged with: