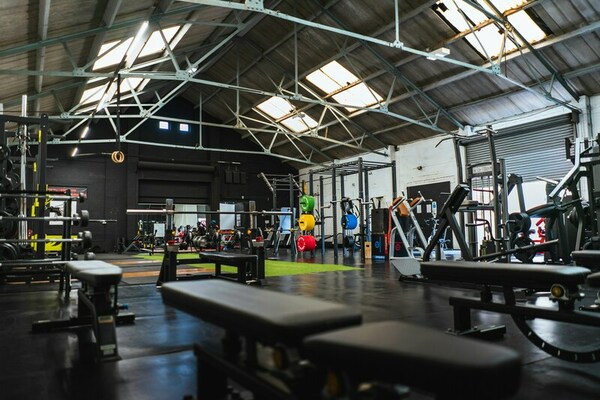
Here the authors investigated the relationship between fitness-related social media and the high usage of performance-enhancing drugs (PEDs) specifically by men in the US age 18-35. In a survey with 149 participants they identified that young men that use fitness-related social media are more likely to use PEDs. Their results suggest the necessity to consider potential risk behaviors which may be related to social media consumption.
Read More...