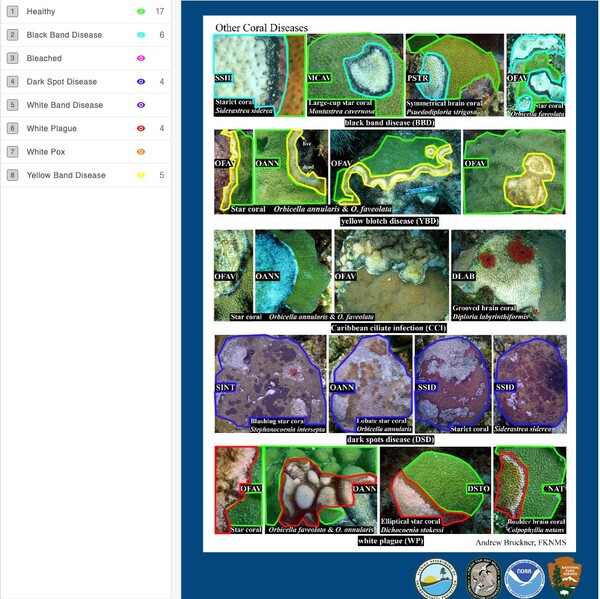
Triggered largely by the warming and pollution of oceans, corals are experiencing bleaching and a variety of diseases caused by the spread of bacteria, fungi, and viruses. Identification of bleached/diseased corals enables implementation of measures to halt or retard disease. Benthic cover analysis, a standard metric used in large databases to assess live coral cover, as a standalone measure of reef health is insufficient for identification of coral bleaching/disease. Proposed herein is a solution that couples machine learning with crowd-sourced data – images from government archives, citizen science projects, and personal images collected by tourists – to build a model capable of identifying healthy, bleached, and/or diseased coral.
Read More...