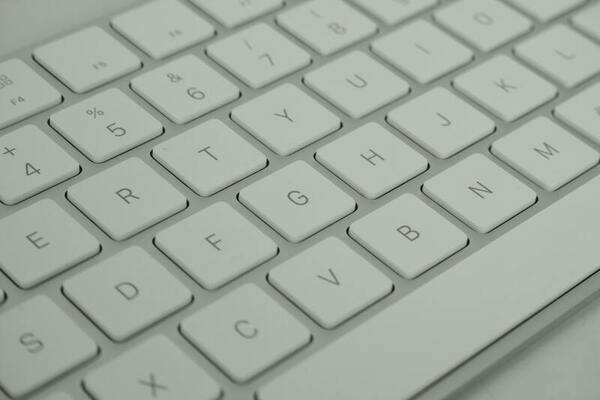
In cognitive psychology, typed responses are used to assess thinking skills and creativity, but research on factors influencing typing speed is limited. This study examined how language familiarity affects typing speed, hypothesizing that familiarity with a language would correlate with faster typing. Participants typed faster in English than Latin, with those unfamiliar with Latin showing a larger discrepancy between the two languages, though Latin education level did not significantly impact typing speed, highlighting the role of language familiarity in typing performance.
Read More...