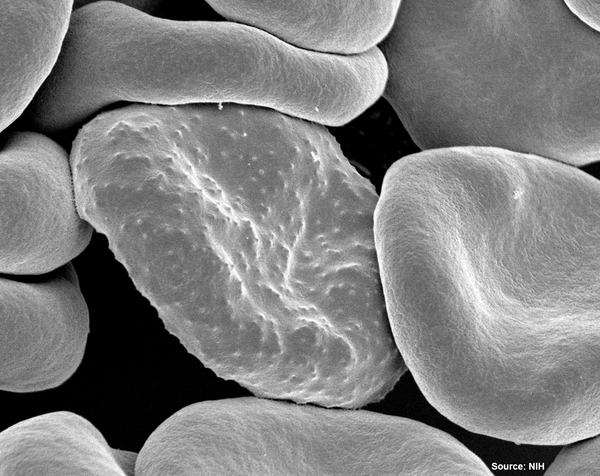
The diagnosis of malaria remains one of the major hurdles to eradicating the disease, especially among poorer populations. Here, the authors use machine learning to improve the accuracy of deep learning algorithms that automate the diagnosis of malaria using images of blood smears from patients, which could make diagnosis easier and faster for many.
Read More...