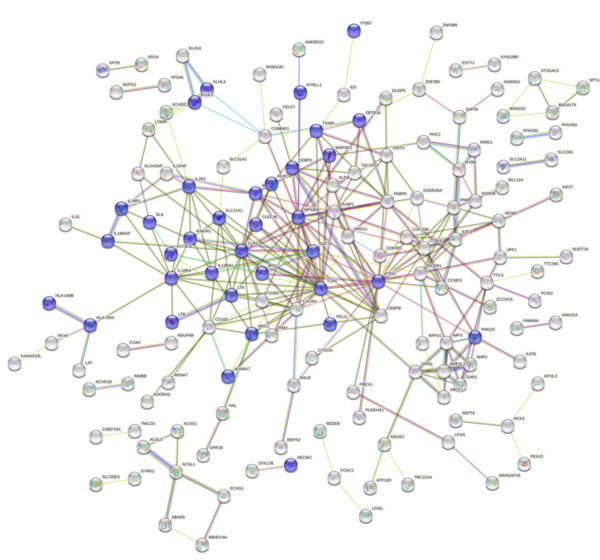
Major depressive disorder (MDD) is a prevalent mood disorder. The direct causes and biological mechanisms of depression still elude understanding, though genetic factors have been implicated. This study looked to identify the mechanism behind the aberrant response to the dexamethasone suppression test (DST) displayed by MDD patients, in which they display a lack of cortisol suppression. Analysis revealed several pro-inflammatory genes that were significant and differentially expressed between affected and non-affected groups in response to the DST. Looking at ways to decrease the inflammatory response could have implications for treatment and may explain why some people treated for depression still display symptoms or may lead researchers to different classes of drugs for treatment.
Read More...