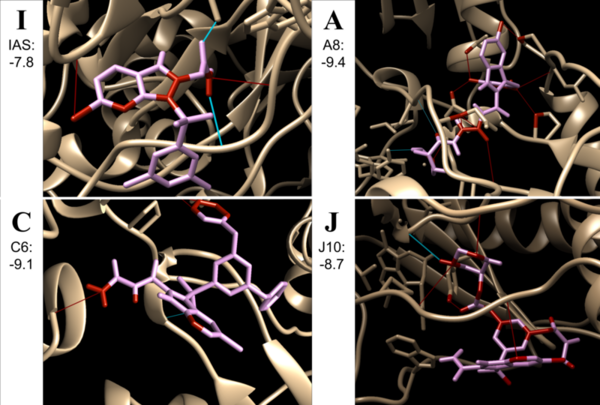
Non-nucleoside reverse transcriptase inhibitors (NNRTIs) are allosteric inhibitors that bind to the HIV reverse transcriptase and prevent replication. Indolyl aryl sulfones (IAS) and IAS derivatives have been found to be highly effective against mutant strains of HIV-1 reverse transcriptase. Here, we analyzed molecules designed using aryl sulfone scaffolds paired to cyclic compounds as potential NNRTIs through the computational design and docking of 100 novel NNRTI candidates. Moreover, we explored the specific combinations of functional groups and aryl sulfones that resulted in the NNRTI candidates with the strongest binding affinity while testing all compounds for carcinogenicity. We hypothesized that the combination of an IAS scaffold and pyrimidine would produce the compounds with the best binding affinity. Our hypothesis was correct as the series of molecules with an IAS scaffold and pyrimidine exhibited the best average binding affinity. Additionally, this study found 32 molecules designed in this procedure with higher or equal binding affinities to the previously successful IAS derivative 5-bromo-3-[(3,5-dimethylphenyl)sulfonyl]indole-2-carboxyamide when docked to HIV-1 reverse transcriptase.
Read More...