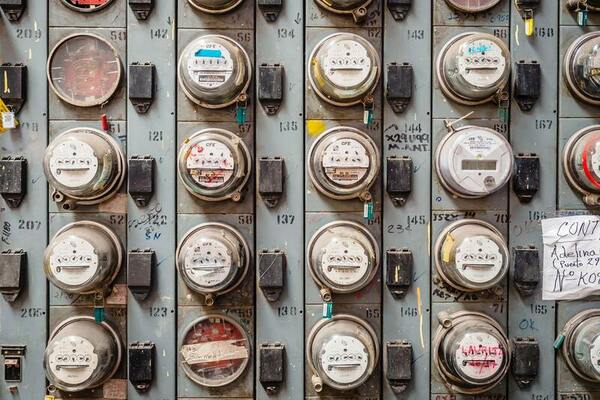
The authors found that SARIMAX-GARCH is more accurate than SARIMAX for load forecasting with respect to energy consumption.
Read More...An analysis of the feasibility of SARIMAX-GARCH through load forecasting
The authors found that SARIMAX-GARCH is more accurate than SARIMAX for load forecasting with respect to energy consumption.
Read More...Differential privacy in machine learning for traffic forecasting
In this paper, we measured the privacy budgets and utilities of different differentially private mechanisms combined with different machine learning models that forecast traffic congestion at future timestamps. We expected the ANNs combined with the Staircase mechanism to perform the best with every value in the privacy budget range, especially with the medium high values of the privacy budget. In this study, we used the Autoregressive Integrated Moving Average (ARIMA) and neural network models to forecast and then added differentially private Laplacian, Gaussian, and Staircase noise to our datasets. We tested two real traffic congestion datasets, experimented with the different models, and examined their utility for different privacy budgets. We found that a favorable combination for this application was neural networks with the Staircase mechanism. Our findings identify the optimal models when dealing with tricky time series forecasting and can be used in non-traffic applications like disease tracking and population growth.
Read More...Population Forecasting by Population Growth Models based on MATLAB Simulation
In this work, the authors investigate the accuracy with which two different population growth models can predict population growth over time. They apply the Malthusian law or Logistic law to US population from 1951 until 2019. To assess how closely the growth model fits actual population data, a least-squared curve fit was applied and revealed that the Logistic law of population growth resulted in smaller sum of squared residuals. These findings are important for ensuring optimal population growth models are implemented to data as population forecasting affects a country's economic and social structure.
Read More...Bacterial Load Consistency Among Three Independent Water Distribution Systems
Clean drinking water is an essential component to maintaining public health. The authors of this study tested the bacterial load of water from three different disinfection and filtration systems in order to determine which system might be superior.
Read More...A novel encoding technique to improve non-weather-based models for solar photovoltaic forecasting
Several studies have applied different machine learning (ML) techniques to the area of forecasting solar photovoltaic power production. Most of these studies use weather data as inputs to predict power production; however, there are numerous practical issues with the procurement of this data. This study proposes models that do not use weather data as inputs, but rather use past power production data as a more practical substitute to weather-based models. Our proposed models demonstrate a better, cheaper, and more reliable alternatives to current weather models.
Read More...COVID-19 and air pollution in New York City
Did the COVID-19 pandemic and travel restrictions improve air quality? The authors investigate this question in New York City using existing pollution data and forecasting trends.
Read More...A study on the stretching behavior of rubber bands
Here, the authors considered the stretching behavior of rubber bands by exposing the rubber bands to increasing loads and measuring their stretch response. They found that a linear stretch response was observed for intermediate loading steps, but this behavior was lost at lower or higher loads, deviating from Hooke's Law. The authors suggest that studies such as these can be used to evaluate other visco-elastic structures.
Read More...Testing Epoxy Strength: The High Strength Claims of Selleys’s Araldite Epoxy Glues
Understanding the techniques used to improve the adhesion strength of the epoxy resin is important especially for consumer applications such as repairing car parts, bonding aluminum sheeting, and repairing furniture or applications within the aviation or civil industry. Selleys Araldite epoxy makes specific strength claims emphasizing that the load or weight that can be supported by the adhesive is 72 kg/cm2. Nguyen and Clarke aimed to test the strength claims of Selley’s Araldite Epoxy by gluing two steel adhesion surfaces: a steel tube and bracket. Results showed that there is a lack of consideration by Selleys for adhesion loss mechanisms and environmental factors when accounting for consumer use of the product leading to disputable claims.
Read More...In silico modeling of emodin’s interactions with serine/threonine kinases and chitosan derivatives
Here, through protein-ligand docking, the authors investigated the effect of the interaction of emodin with serine/threonine kinases, a subclass of kinases that is overexpressed in many cancers, which is implicated in phosphorylation cascades. Through molecular dynamics theyfound that emodin forms favorable interactions with chitosan and chitosan PEG (polyethylene glycol) copolymers, which could aid in loading drugs into nanoparticles (NPs) for targeted delivery to cancerous tissue. Both polymers demonstrated reasonable entrapment efficiencies, which encourages experimental exploration of emodin through targeted drug delivery vehicles and their anticancer activity.
Read More...Using data science along with machine learning to determine the ARIMA model’s ability to adjust to irregularities in the dataset
Auto-Regressive Integrated Moving Average (ARIMA) models are known for their influence and application on time series data. This statistical analysis model uses time series data to depict future trends or values: a key contributor to crime mapping algorithms. However, the models may not function to their true potential when analyzing data with many different patterns. In order to determine the potential of ARIMA models, our research will test the model on irregularities in the data. Our team hypothesizes that the ARIMA model will be able to adapt to the different irregularities in the data that do not correspond to a certain trend or pattern. Using crime theft data and an ARIMA model, we determined the results of the ARIMA model’s forecast and how the accuracy differed on different days with irregularities in crime.
Read More...Search articles by title, author name, or tags