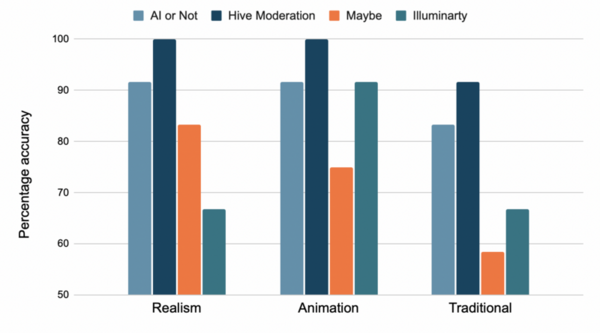
The authors investigate how well AI-detection machine learning models can detect real versus AI-generated art across different art styles.
Read More...Evaluating the effectiveness of machine learning models for detecting AI-generated art
The authors investigate how well AI-detection machine learning models can detect real versus AI-generated art across different art styles.
Read More...Vineyard vigilance: Harnessing deep learning for grapevine disease detection
Globally, the cultivation of 77.8 million tons of grapes each year underscores their significance in both diets and agriculture. However, grapevines face mounting threats from diseases such as black rot, Esca, and leaf blight. Traditional detection methods often lag, leading to reduced yields and poor fruit quality. To address this, authors used machine learning, specifically deep learning with Convolutional Neural Networks (CNNs), to enhance disease detection.
Read More...SpottingDiffusion: Using transfer learning to detect Latent Diffusion Model-synthesized images
An explainable model for content moderation
The authors looked at the ability of machine learning algorithms to interpret language given their increasing use in moderating content on social media. Using an explainable model they were able to achieve 81% accuracy in detecting fake vs. real news based on language of posts alone.
Read More...Artificial intelligence assisted violin performance learning
In this study the authors looked at the ability of artificial intelligence to detect tempo, rhythm, and intonation of a piece played on violin. Technology such as this would allow for students to practice and get feedback without the need of a teacher.
Read More...Environmentally-friendly graphene conductive ink using graphene powder, polystyrene, and waste oil
In this article, the authors propose an effective, environmentally-friendly method of producing conductive ink using expired waste oil, polystyrene, and graphene.
Read More...Unveiling bias in ChatGPT-3.5: Analyzing constitutional AI principles for politically biased responses
Various methods exist to mitigate bias in AI models, including "Constitutional AI," a technique which guides the AI to behave according to a list of rules and principles. Lo, Poosarla, Singhal, Li, Fu, and Mui investigate whether constitutional AI can reduce bias in AI outputs on political topics.
Read More...Comparing model-centric and data-centric approaches to determine the efficiency of data-centric AI
In this study, three models are used to test the hypothesis that data-centric artificial intelligence (AI) will improve the performance of machine learning.
Read More...Trust in the use of artificial intelligence technology for treatment planning
As AI becomes more integrated into healthcare, public trust in AI-developed treatment plans remains a concern, especially for emotionally charged health decisions. In a study of 81 community college students, AI-created treatment plans received lower trust ratings compared to physician-developed plans, supporting the hypothesis. The study found no significant differences in AI trust levels across demographic factors, suggesting overall skepticism toward AI-driven healthcare.
Read More...Propagation of representation bias in machine learning
Using facial recognition as a use-case scenario, we attempt to identify sources of bias in a model developed using transfer learning. To achieve this task, we developed a model based on a pre-trained facial recognition model, and scrutinized the accuracy of the model’s image classification against factors such as age, gender, and race to observe whether or not the model performed better on some demographic groups than others. By identifying the bias and finding potential sources of bias, his work contributes a unique technical perspective from the view of a small scale developer to emerging discussions of accountability and transparency in AI.
Read More...