SpottingDiffusion: Using transfer learning to detect Latent Diffusion Model-synthesized images
(1) The Westminster School, Dubai
https://doi.org/10.59720/23-256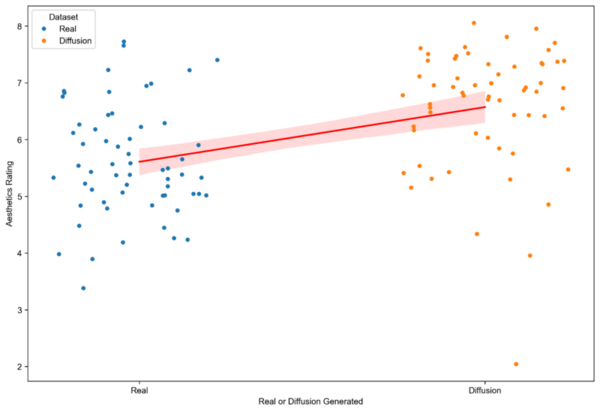
Differentiating images made by Artificial Intelligence (AI) from real ones has recently become an issue of great importance as realistic AI-generated images rapidly become easier to make and disseminate. Our study aims to present a novel approach for detecting images produced by Latent Diffusion Models (LDMs). The need to detect these images arises when these technologies are used to make images which can mislead the human viewer. We present a solution: an algorithmic way of differentiating images made by LDMs from real ones. In particular, we detail our research on detecting images produced by the Stable Diffusion LDM. We hypothesized that our model could differentiate AI-generated images from real images more accurately than three other recently created methods which aim to do the same. Our transfer learning-based approach to detect LDM-generated images successfully learned to recognize images made by the Stable Diffusion LDM and had a 62.5% accuracy on our testing dataset of LDM-generated images from online, real-world sources. We tested our Transfer Learning-based approach, SpottingDiffusion, against three other recent methods. The best of these methods achieved an accuracy of up to 50.8% on our testing dataset, while SpottingDiffusion achieved an accuracy of 62.5%. These results supported our hypothesis that SpottingDiffusion is more accurate than the other tested methods.
This article has been tagged with: