Depression detection in social media text: leveraging machine learning for effective screening
(1) Asia Pacific International School (APIS), (2) Fermi National Accelerator Laboratory
https://doi.org/10.59720/24-008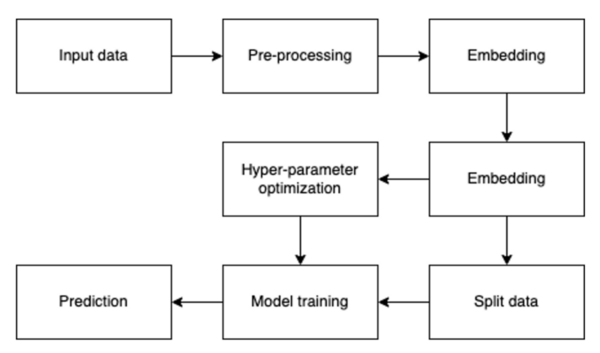
Depression is a significant mental health concern that affects millions of people worldwide. Early diagnosis and intervention are crucial for effective treatment. However, identifying depression symptoms can often be challenging. From the current new wave of social media platforms, we hypothesized that examining texts in social media is an enabling pathway to describe a person’s mental condition, compared to conventional protocol applied in clinical diagnosis. In this study, we investigated the detection of depression-related patterns on social media platforms. We utilized advanced classification algorithms in order to extract key linguistic features and emotional expressions from social media texts. By applying two supervised classification machine learning algorithms, decision trees and random forests, we were able to distinguish differences between the depression and non-depression conditions. We applied natural language processing, an AI-powered language model which is the basis of ChatGPT. Our study suggests that screening mental disorders is feasible only by looking at texts. Hence, we suppose that our developed method would be applicable to evaluate mental disorders by leveraging a person's activity level. It is also expected that this way would further improve the accuracy of depression diagnosis by considering both physical and psychological symptoms.
This article has been tagged with: