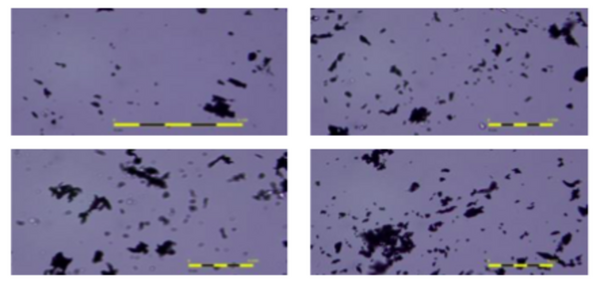
In this article, the authors propose an effective, environmentally-friendly method of producing conductive ink using expired waste oil, polystyrene, and graphene.
Read More...Environmentally-friendly graphene conductive ink using graphene powder, polystyrene, and waste oil
In this article, the authors propose an effective, environmentally-friendly method of producing conductive ink using expired waste oil, polystyrene, and graphene.
Read More...Developing a neural network to model the mechanical properties of 13-8 PH stainless steel alloy
We systematically evaluated the effects of raw material composition, heat treatment, and mechanical properties on 13-8PH stainless steel alloy. The results of the neural network models were in agreement with experimental results and aided in the evaluation of the effects of aging temperature on double shear strength. The data suggests that this model can be used to determine the appropriate 13-8PH alloy aging temperature needed to achieve the desired mechanical properties, eliminating the need for many costly trials and errors through re-heat treatments.
Read More...The effect of activation function choice on the performance of convolutional neural networks
With the advance of technology, artificial intelligence (AI) is now applied widely in society. In the study of AI, machine learning (ML) is a subfield in which a machine learns to be better at performing certain tasks through experience. This work focuses on the convolutional neural network (CNN), a framework of ML, applied to an image classification task. Specifically, we analyzed the performance of the CNN as the type of neural activation function changes.
Read More...A novel filtration model for microplastics using natural oils and its application to the environment
Recognizing the need for a method to filter microplastics from polluted water the authors sought to use nonpolar solvents, palm oil and palm kernel oil, to filter microplastics out of model seawater. By relying on the separation of polar and nonpolar solvents followed by freezing the nonpolar solvent, they reported that microplastics could be extracted with percentages ranging from 96.2% to 94.2%. They also provided an estimation to use this method as part of container ships to clean the Pacific Ocean of microplastics.
Read More...A Temperature-Based Comparison of Compounds Found in Bao Chong Tea, Green Tea, and Black Tea
While tea has a complex history, recently the health benefits of this beverage have come into focus. In this study, researchers sought to compare the levels of caffeine, catechins and L-theanine between different types of tea using NMR spectroscopy. Further, the impact of brewing temperature on the release of these compounds was also assessed. Of those tested, Bao Chong tea had the highest levels of these compounds. Brewing temperatures between 45ºC and 75ºC were found to be optimal for compound release. These results can help consumers make informed choices about their tea preparation and intake.
Read More...Differences in Reliability and Predictability of Harvested Energy from Battery-less Intermittently Powered Systems
Solar and radio frequency harvesters serve as a viable alternative energy source to batteries in many cases where the battery cannot be easily replaced. Using specifically designed circuit models, the authors quantify the reliability of different harvested energy sources to identify the most practical and efficient forms of renewable energy.
Read More...Changes for Development of Al2O3 Coated PVA (Polyvinyl Alcohol) Composite Nonwoven Separator For Improving Thermal and Electrochemical Properties
Lithium-ion batteries, a breakthrough in chemistry that enabled the electronic revolution we live today have become an essential part of our day-to-day life. A phone battery running out after a heavy day of use with limited opportunities for recharging is a well-known and resented experience by almost everyone. How then can we make batteries more efficient? This paper proposes the use of a different type of separator, that improves the charging and discharging capacities of lithium ions compared to the classical separator. This and similar attempts to improve Lithium-ion battery function could facilitate the development of higher-performance batteries that work longer and withstand harsher use.
Read More...Methanotrophic bioremediation for the degradation of oceanic methane and chlorinated hydrocarbons
Seeking an approach to address the increasing levels of methane and chlorinated hydrocarbons that threaten the environment, the authors worked to develop a novel, low-cost biotrickling filter for use as an ex situ method tailored to marine environments. By using methanotrophic bacteria in the filter, they observed methane degradation, suggesting the feasibility of chlorinated hydrocarbon degradation.
Read More...Fabrication of CuSbS2 Solar Cells by Sulfurization of Thermally Evaporated Metal Stacks
In this article, the authors created CuSbS2 solar cells. They discovered that the cells' efficiency was affected by the formation of MoS2. By incorporating a layer of single-walled carbon nanotubes, however, they were able to prevent MoS2 formation and increase the device's efficiency.
Read More...The optical possibilities of gelatin
Here the authors investigated the optical possibilities of gelatin and acrylic in regards to potential implementations at soft contact lenses. They fabricated lenses of different shapes and evaluated the refraction of laser light finding that gelatin needed to be thickened or increased in curvature to account for its lower refractive index compared to plastics, or used in a mixture to strengthen the lens.
Read More...Search articles by title, author name, or tags