Convolutional neural network-based analysis of pediatric chest X-ray images for pneumonia detection
(1) Santa Catalina School, (2) Radcliffe Department of Medicine, University of Oxford
https://doi.org/10.59720/24-031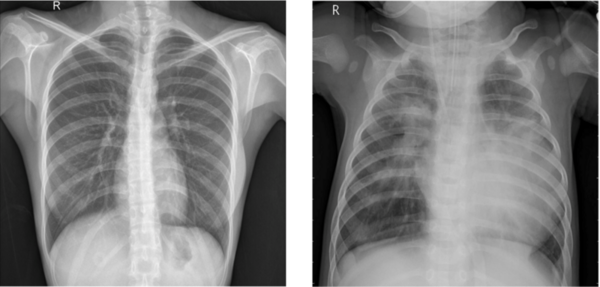
Detection of pneumonia is generally done using chest X-ray images, traditionally assessed manually by radiologists. However, this method has limitations, including the potential for human error, variability in interpretation, and a shortage of skilled professionals, particularly in resource-limited settings. Prompted by these challenges and the increasing potential of machine learning in medical diagnostics, we investigated the efficacy of advanced computational models in distinguishing between normal and pneumonia-affected lung images. We hypothesized that an adapted version of the VGG16 model, a convolutional neural network (CNN), would outperform the standard VGG16 and simpler Multilayer Perceptrons (MLPs) in terms of accuracy and reliability. Utilizing a dataset from the Guangzhou Women and Children’s Medical Center, we evaluated the performance of these three models on pediatric chest X-ray images. The MLP showed moderate effectiveness with 78.4% accuracy but struggled with complex image data. The standard VGG16 achieved better results with 90.9% accuracy but displayed overfitting tendencies. The adapted VGG16 model, with reduced filter sizes and dropout layers, demonstrated the highest accuracy at 95.6%, indicating superior performance and stability. These findings suggest that tailored deep learning models like the adapted VGG16 can significantly enhance pneumonia diagnosis from chest X-ray images, offering a balance of accuracy, efficiency, and generalizability. This advancement holds substantial implications for improving diagnostic processes in pediatric healthcare, particularly in settings with limited resources.
This article has been tagged with: