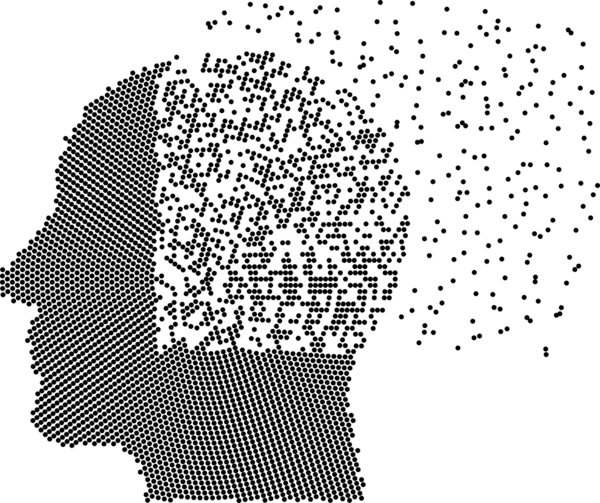
Alzheimer's disease (AD) involves the reduction of cholinergic activity due to a decrease in neuronal levels of nAChR α7. In this work, Sanyal and Cuellar-Ortiz explore the role of the nAChR α7 in learning and memory retention, using Drosophila melanogaster as a model organism. The performance of mutant flies (PΔEY6) was analyzed in locomotive and olfactory-memory retention tests in comparison to wild type (WT) flies and an Alzheimer's disease model Arc-42 (Aβ-42). Their results suggest that the lack of the D. melanogaster-nAChR causes learning, memory, and locomotion impairments, similar to those observed in Alzheimer's models Arc-42.
Read More...