Machine learning predictions of additively manufactured alloy crack susceptibilities
(1) Basis Independent Fremont, (2) Monta Vista High School, (3) Valley Christian High School, (4) Department of Materials, University of California, Santa Barbara
https://doi.org/10.59720/24-057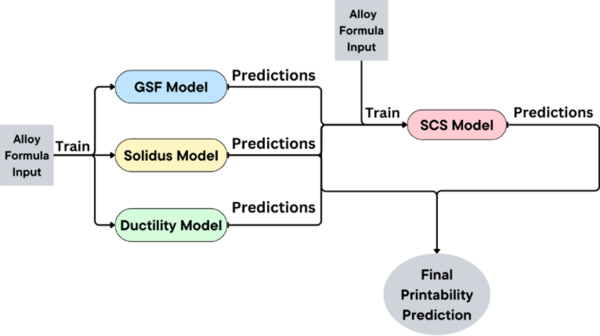
Additive manufacturing (AM) is revolutionizing the production of complex metal parts, offering both extreme precision and cost reduction. However, structural defects such as internal cracking can pose serious issues in AM parts, especially in safety-critical applications such as aerospace and automotive production. Empirically testing or running high-throughput simulations on a high volume of alloy formulas to quantify these cracking susceptibilities is overly costly and time-consuming. Recent developments in machine learning (ML) offer a more efficient solution to predicting the properties of a high volume of materials without the time cost needed to run mechanical simulations. In this study, we analyzed the solidification cracking susceptibilities (SCS) of potential printing alloys in safety-critical, additively manufactured parts. Based on the link between SCS and secondary alloy properties, we hypothesized that a multi-model ML pipeline would predict an alloy’s solidification cracking susceptibility at a higher accuracy than a model trained on alloy composition alone. Since predicting SCS directly from alloy composition eliminates the need for empirical measurements, our pipeline also predicted these secondary attributes directly from the original composition. We found that a pipeline using Random Forest architecture models performed at the highest accuracy and precision, outperforming the precision of a random forest model with no secondary predictions. Furthermore, the results of this study indicated a correlation between SCS and the included secondary attributes, which can be investigated in further research, as these attributes have not yet been factored into current SCS quantification methods.
This article has been tagged with: