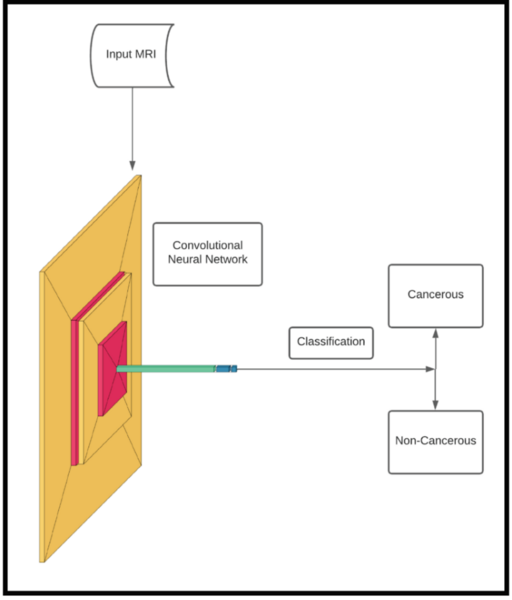
Using a convolution neural network, these authors show machine learning can clinically diagnose breast cancer with high accuracy.
Read More...Predicting the Instance of Breast Cancer within Patients using a Convolutional Neural Network
Using a convolution neural network, these authors show machine learning can clinically diagnose breast cancer with high accuracy.
Read More...Tomato disease identification with shallow convolutional neural networks
Plant diseases can cause up to 50% crop yield loss for the popular tomato plant. A mobile device-based method to identify diseases from photos of symptomatic leaves via computer vision can be more effective due to its convenience and accessibility. To enable a practical mobile solution, a “shallow” convolutional neural networks (CNNs) with few layers, and thus low computational requirement but with high accuracy similar to the deep CNNs is needed. In this work, we explored if such a model was possible.
Read More...Transfer Learning with Convolutional Neural Network-Based Models for Skin Cancer Classification
Skin cancer is a common and potentially deadly form of cancer. This study’s purpose was to develop an automated approach for early detection for skin cancer. We hypothesized that convolutional neural network-based models using transfer learning could accurately differentiate between benign and malignant moles using natural images of human skin.
Read More...Determining the best convolutional neural network for identifying tuberculosis and pneumonia in chest x-rays
To best identify tuberculosis and pneumonia diagnoses in chest x-rays, the authors compare different deep learning convolution neural networks.
Read More...The effect of activation function choice on the performance of convolutional neural networks
With the advance of technology, artificial intelligence (AI) is now applied widely in society. In the study of AI, machine learning (ML) is a subfield in which a machine learns to be better at performing certain tasks through experience. This work focuses on the convolutional neural network (CNN), a framework of ML, applied to an image classification task. Specifically, we analyzed the performance of the CNN as the type of neural activation function changes.
Read More...The Effect of Varying Training on Neural Network Weights and Visualizations
Neural networks are used throughout modern society to solve many problems commonly thought of as impossible for computers. Fountain and Rasmus designed a convolutional neural network and ran it with varying levels of training to see if consistent, accurate, and precise changes or patterns could be observed. They found that training introduced and strengthened patterns in the weights and visualizations, the patterns observed may not be consistent between all neural networks.
Read More...Augmented Reality Chess Analyzer (ARChessAnalyzer): In-Device Inference of Physical Chess Game Positions through Board Segmentation and Piece Recognition using Convolutional Neural Networks
In this study the authors develop an app for faster chess game entry method to help chess learners improve their game. This culminated in the Augmented Reality Chess Analyzer (ARChessAnalyzer) which uses traditional image and vision techniques for chess board recognition and Convolutional Neural Networks (CNN) for chess piece recognition.
Read More...Enhancing marine debris identification with convolutional neural networks
Plastic pollution in the ocean is a major global concern. Remotely Operated Vehicles (ROVs) have promise for removing debris from the ocean, but more research is needed to achieve full effectiveness of the ROV technology. Wahlig and Gonzales tackle this issue by developing a deep learning model to distinguish trash from the environment in ROV images.
Read More...Identifying Neural Networks that Implement a Simple Spatial Concept
Modern artificial neural networks have been remarkably successful in various applications, from speech recognition to computer vision. However, it remains less clear whether they can implement abstract concepts, which are essential to generalization and understanding. To address this problem, the authors investigated the above vs. below task, a simple concept-based task that honeybees can solve, using a conventional neural network. They found that networks achieved 100% test accuracy when a visual target was presented below a black bar, however only 50% test accuracy when a visual target was presented below a reference shape.
Read More...DNA-SEnet: A convolutional neural network for classifying DNA-asthma associations
In this study, the authors developed a model named DNA Sequence Embedding Network (DNA-SEnet) to classify DNA-asthma associations using their genomic patterns.
Read More...Search articles by title, author name, or tags