DNA-SEnet: A convolutional neural network for classifying DNA-asthma associations
(1) BASIS Scottsdale, Scottsdale, Arizona, (2) Stanford University Department of Bioengineering, Stanford, California
https://doi.org/10.59720/21-099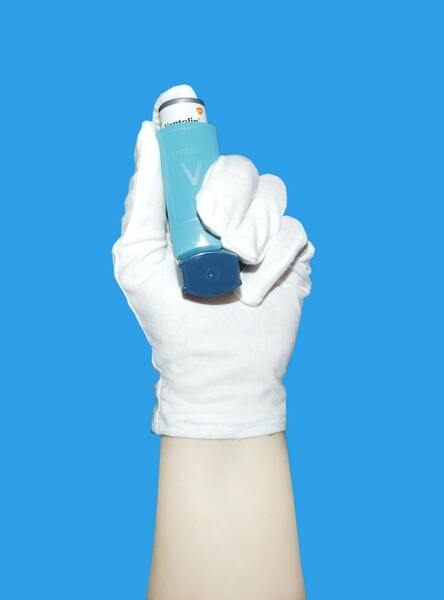
Asthma is a complex disease with a growing global prevalence whose genetic causes remain largely unexplored. The rise of next-generation sequencing has significantly augmented genetic studies in identifying asthma-associated mutations, the most common of which are single nucleotide polymorphisms (SNPs). Population-based and biochemical analyses have been used to identify novel disease-associated loci and their biological consequences; however, SNPs alone do not explain the mechanisms of asthma nor do they offer a context to evaluate candidate SNP-asthma associations. To this end, we developed a model named DNA Sequence Embedding Network (DNA-SEnet) to classify DNA-asthma associations using their genomic patterns. The hypotheses of this study are that DNA-asthma associations can be discerned through high-dimensional vector representations of DNA sequences around SNPs, that these features can be applied to determine novel SNP-asthma associations, and that this model can be generalized to predict SNP-disease associations for other complex traits. On average, this model achieved an Area Under the Curve (AUC) equaling 0.81 when learning and classifying DNA-asthma associations. Additionally, DNA-SEnet corroborated previous studies’ SNP-asthma connections and proposed two novel asthma-linked loci based on their surrounding semantic properties. Moreover, DNA-SEnet effectively learned DNA-disease associations when applied to sequence data regarding coronary heart disease, type 2 diabetes mellitus, and rheumatoid arthritis. Therefore, this model can be used to identify novel disease-associated sequences across various disease types.
This article has been tagged with: