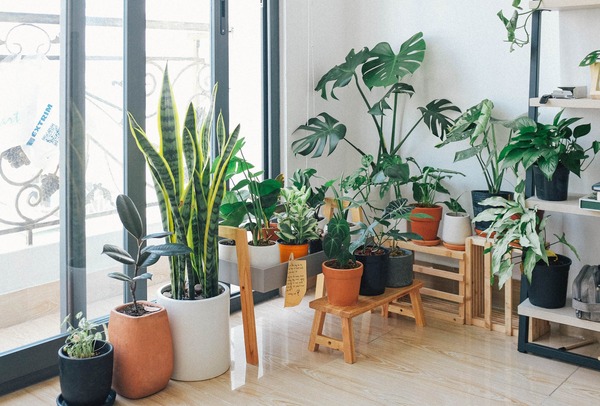
In this report, Bhardwaj and Sharma tested whether placing specific plants indoors can reduce levels of indoor air pollution that can lead to lung-related illnesses. Using machine learning, they show that plants improved overall indoor air quality and reduced levels of particulate matter. They suggest that plant-based interventions coupled with sensors may be a useful long-term solution to reducing and maintaining indoor air pollution.
Read More...