Identifying Neural Networks that Implement a Simple Spatial Concept
(1) River Hill High School, Clarksville, Maryland, (2) Center for Theoretical Neuroscience, Columbia University, New York City, New York
https://doi.org/10.59720/22-046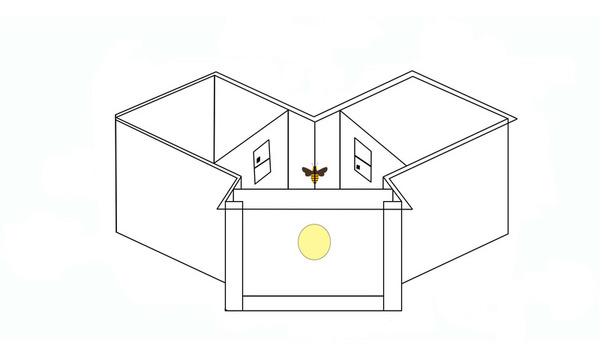
Modern artificial neural networks have been remarkably successful in various applications, from speech recognition to computer vision. However, it remains less clear whether they can implement abstract concepts, which are essential to generalization and understanding. To address this problem, we investigated the above vs. below task, a simple concept-based task that honeybees can solve. We hypothesized that neural networks would successfully solve this task, and that performance would vary substantially between network architectures. Specifically, we predicted that the convolutional neural network (CNN), a prototypical architecture well known for its ability to classify objects accurately, would perform better than the single-layer and multi-layer perceptrons (SLP and MLP, respectively). In the first task (Experiment 1), a visual target was presented above or below a black bar; in the second (Experiment 2), a visual target was presented above or below a reference shape. We found that all networks achieved 100% testing accuracy on Experiment 1. In contrast, the networks’ accuracy differed substantially in Experiment 2. The SLP had only a 50% testing accuracy, and the CNN outperformed the MLP (98% vs. 81% accuracy, respectively). Further analysis of the connection weights and distances between shapes suggested that the MLP may not evaluate relative spatial relationships in Experiment 2. Instead, the network seemed to partition the image into upper and lower zones, which appears inconsistent with the concept of relative locations. These findings indicate different capacities of network architectures, offer insight into their mechanisms, and motivate work on how neural systems implement conceptual knowledge.
This article has been tagged with: