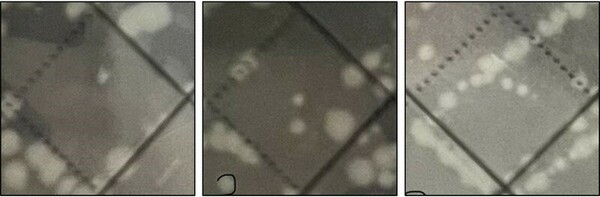
The authors test the effects of the pesticide captan on the growth of gut microbiome bacteria including Bacillus coagulan and Escherichia coli.
Read More...Inhibitory effects of captan on growth of Escherichia coli and Bacillus coagulans
The authors test the effects of the pesticide captan on the growth of gut microbiome bacteria including Bacillus coagulan and Escherichia coli.
Read More...Artificial intelligence assisted violin performance learning
In this study the authors looked at the ability of artificial intelligence to detect tempo, rhythm, and intonation of a piece played on violin. Technology such as this would allow for students to practice and get feedback without the need of a teacher.
Read More...Evaluating machine learning algorithms to classify forest tree species through satellite imagery
Here, seeking to identify an optimal method to classify tree species through remote sensing, the authors used a few machine learning algorithms to classify forest tree species through multispectral satellite imagery. They found the Random Forest algorithm to most accurately classify tree species, with the potential to improve model training and inference based on the inclusion of other tree properties.
Read More...The effects of UV-C and ionizing radiation on the functions of Escherichia Coli
In this study, the authors send E. coli cultures to space via the Cubes in SpaceTM program to determine if ultraviolet C and ionizing radiation negatively affect bacterial growth.
Read More...Heavy Metal Contamination of Hand-Pressed Well Water in HuNan, China
Unprocessed water from hand-pressed wells is still commonly used as a source of drinking water in Chenzhou, the “Nonferrous Metal Village” of China. Long et al. conducted a study to measure the heavy metal contamination levels and potential health effects in this area. Water samples were analyzed through Inductively Coupled Plasma Optical Emission Spectroscopy (ICPOES) and the concentrations of 20 metal elements. Results showed that although none of the samples had dangerous levels of heavy metals, the concentrations of Al, Fe, and Mn in many locations substantially exceeded those suggested in the Chinese Drinking Water Standard and the maximum contaminant levels of Environmental Protection Agency (EPA). The authors have made an important discovery regarding the water safety in HuNan and their suggestions to install water treatment systems would greatly benefit the community.
Read More...Predicting asthma-related emergency department visits and hospitalizations with machine learning techniques
Seeking to investigate the effects of ambient pollutants on human respiratory health, here the authors used machine learning to examine asthma in Lost Angeles County, an area with substantial pollution. By using machine learning models and classification techniques, the authors identified that nitrogen dioxide and ozone levels were significantly correlated with asthma hospitalizations. Based on an identified seasonal surge in asthma hospitalizations, the authors suggest future directions to improve machine learning modeling to investigate these relationships.
Read More...