Determining viability of image processing models for forensic analysis of hair for related individuals
(1) Tesla STEM High School, (2) University of Central Florida Department of Chemistry
https://doi.org/10.59720/24-032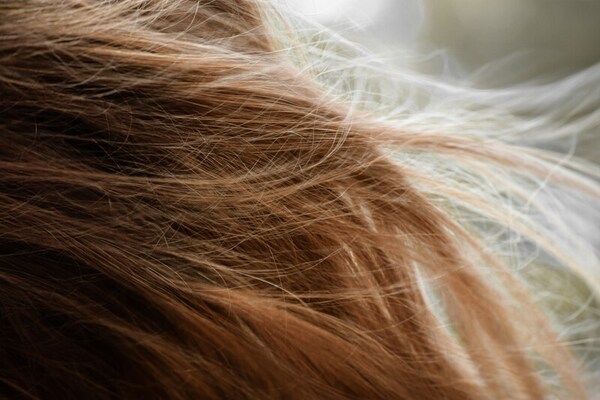
Errors in testimonies of microscopical hair examination have led to dozens of false convictions, since hair strands from different people can look similar. Mitochondrial DNA (mtDNA) can be obtained from hairs; however, because mtDNA is identical along the maternal line, it is ineffective in distinguishing between family members. While nuclear DNA (nDNA) can be extracted from the hair’s root, it is not always present, and nDNA in the hair shaft is limited and often degraded. This limitation has led to extensive research on objective methods of differentiating individuals using microscopical imaging and statistical analysis. We aimed to determine if there was a machine learning algorithm with the highest accuracy in individual classification using imaging of microscopical analysis of hair. Images of 644 hair sections were analyzed by objectively quantifying the hair’s medulla, width, color, texture, and pigment distribution. To determine the highest accuracy, we used four supervised models: discriminant analysis (DA), multinomial logistic regression, K-nearest neighbor, and support vector machine; and two unsupervised models: principal component analysis and hierarchical cluster analysis. The accuracy was tested on isolated data from nine families to test cases where mtDNA would fall short in differentiating related individuals. Unlike the unsupervised models, the supervised models provided comprehensive results of accuracy determination. The DA model yielded the highest accuracy of 88.89%, with 3/5 families with twins meeting or exceeding this accuracy. Our results demonstrated DA as the strongest statistical model for future research in differentiating between related individuals, which may increase the probative value of the evidence.
This article has been tagged with: