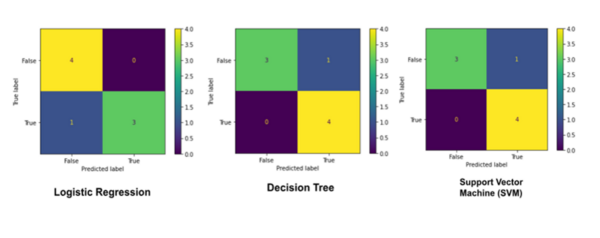
The authors trained a machine learning model to detect kidney stones based on characteristics of urine. This method would allow for detection of kidney stones prior to the onset of noticeable symptoms by the patient.
Read More...A machine learning approach to detect renal calculi by studying the physical characteristics of urine
The authors trained a machine learning model to detect kidney stones based on characteristics of urine. This method would allow for detection of kidney stones prior to the onset of noticeable symptoms by the patient.
Read More...Utilizing meteorological data and machine learning to predict and reduce the spread of California wildfires
This study hypothesized that a machine learning model could accurately predict the severity of California wildfires and determine the most influential meteorological factors. It utilized a custom dataset with information from the World Weather Online API and a Kaggle dataset of wildfires in California from 2013-2020. The developed algorithms classified fires into seven categories with promising accuracy (around 55 percent). They found that higher temperatures, lower humidity, lower dew point, higher wind gusts, and higher wind speeds are the most significant contributors to the spread of a wildfire. This tool could vastly improve the efficiency and preparedness of firefighters as they deal with wildfires.
Read More...Development of a novel machine learning platform to identify structural trends among NNRTI HIV-1 reverse transcriptase inhibitors
With advancements in machine learning a large data scale, high throughput virtual screening has become a more attractive method for screening drug candidates. This study compared the accuracy of molecular descriptors from two cheminformatics Mordred and PaDEL, software libraries, in characterizing the chemo-structural composition of 53 compounds from the non-nucleoside reverse transcriptase inhibitors (NNRTI) class. The classification model built with the filtered set of descriptors from Mordred was superior to the model using PaDEL descriptors. This approach can accelerate the identification of hit compounds and improve the efficiency of the drug discovery pipeline.
Read More...Sex differences in confidence and memory
In this work, the authors sought to provide an original experiment to investigate the conflict over whether males or females tend to exhibit greater accuracy or confidence in their memories. By using an online portal to obtain a convenience sample, the authors found that their results suggest that though males tend to be more confident regarding their memories, they may in fact remember fewer details. The authors suggest that these findings merit further research before making systematic changes regarding crime scene recall settings.
Read More...A novel approach for predicting Alzheimer’s disease using machine learning on DNA methylation in blood
Here, recognizing the difficulty associated with tracking the progression of dementia, the authors used machine learning models to predict between the presence of cognitive normalcy, mild cognitive impairment, and Alzheimer's Disease, based on blood DNA methylation levels, sex, and age. With four machine learning models and two dataset dimensionality reduction methods they achieved an accuracy of 53.33%.
Read More...Breast cancer mammographic screening by different guidelines among women of different races/ethnicities
Mammographic screening is a common diagnostic tool for breast cancer among average-risk women. The authors hypothesized that adherence rates for mammographic screening may be lower among minorities (non-Hispanic black (NHB) and Hispanic/Latino) than among non-Hispanic whites (NHW) regardless of the guideline applied. The findings support other studies’ results that different racial/ethnic and socio-demographic factors can affect screening adherence. Therefore, healthcare providers should promote breast cancer screening especially among NHW/Hispanic women and women lacking insurance coverage.
Read More...Machine learning-based enzyme engineering of PETase for improved efficiency in plastic degradation
Here, recognizing the recognizing the growing threat of non-biodegradable plastic waste, the authors investigated the ability to use a modified enzyme identified in bacteria to decompose polyethylene terephthalate (PET). They used simulations to screen and identify an optimized enzyme based on machine learning models. Ultimately, they identified a potential mutant PETases capable of decomposing PET with improved thermal stability.
Read More...Association of depression and suicidal ideation among adults with the use of H2 antagonists
In this study, the authors investigate associations between use of histamine H2 receptor antagonists and suicidal ideation in different age groups.
Read More...Significance of Tumor Growth Modeling in the Behavior of Homogeneous Cancer Cell Populations: Are Tumor Growth Models Applicable to Both Heterogeneous and Homogeneous Populations?
This study follows the process of single-cloning and the growth of a homogeneous cell population in a superficial environment over the course of six weeks with the end goal of showing which of five tumor growth models commonly used to predict heterogeneous cancer cell population growth (Exponential, Logistic, Gompertz, Linear, and Bertalanffy) would also best exemplify that of homogeneous cell populations.
Read More...Population Forecasting by Population Growth Models based on MATLAB Simulation
In this work, the authors investigate the accuracy with which two different population growth models can predict population growth over time. They apply the Malthusian law or Logistic law to US population from 1951 until 2019. To assess how closely the growth model fits actual population data, a least-squared curve fit was applied and revealed that the Logistic law of population growth resulted in smaller sum of squared residuals. These findings are important for ensuring optimal population growth models are implemented to data as population forecasting affects a country's economic and social structure.
Read More...