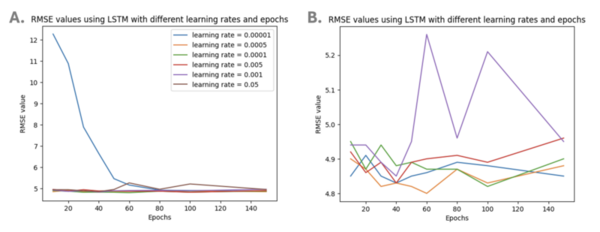
People with Type One diabetes often rely on Continuous Blood Glucose Monitors (CGMs) to track their blood glucose and manage their condition. Researchers are now working to help people with Type One diabetes more easily monitor their health by developing models that will future blood glucose levels based on CGM readings. Jalla and Ghanta tackle this issue by exploring the use of AI models to forecast blood glucose levels with CGM data.
Read More...