Semantic segmentation - labelling each pixel in an image to a specific class- models require large amounts of manually labeled and collected data to train.
Read More...Browse Articles
A Novel Alzheimer's Disease Therapeutic Model: Attenuating Hyperphosphorylated Tau and Amyloid β (Aβ) Aggregates by Characterizing Antioxidative, Anti-Inflammatory, and Neuroprotective Properties of Natural Extracts
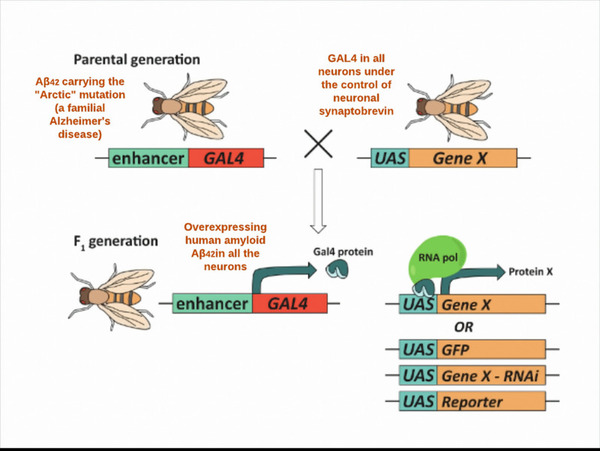
Oxidative damage and neuro-inflammation were the key pathways implicated in the pathogenesis of Alzheimer’s disease. In this study, 30 natural extracts from plant roots and leaves with extensive anti-inflammatory and anti-oxidative properties were consumed by Drosophila melanogaster. Several assays were performed to evaluate the efficacy of these combinational extracts on delaying the progression of Alzheimer’s disease. The experimental group showed increased motor activity, improved associative memory, and decreased lifespan decline relative to the control group.
Read More...Optimizing data augmentation to improve machine learning accuracy on endemic frog calls

The mountain chain of the Western Ghats on the Indian peninsula, a UNESCO World Heritage site, is home to about 200 frog species, 89 of which are endemic. Distinctive to each frog species, their vocalizations can be used for species recognition. Manually surveying frogs at night during the rain in elephant and big cat forests is difficult, so being able to autonomously record ambient soundscapes and identify species is essential. An effective machine learning (ML) species classifier requires substantial training data from this area. The goal of this study was to assess data augmentation techniques on a dataset of frog vocalizations from this region, which has a minimal number of audio recordings per species. Consequently, enhancing an ML model’s performance with limited data is necessary. We analyzed the effects of four data augmentation techniques (Time Shifting, Noise Injection, Spectral Augmentation, and Test-Time Augmentation) individually and their combined effect on the frog vocalization data and the public environmental sounds dataset (ESC-50). The effect of combined data augmentation techniques improved the model's relative accuracy as the size of the dataset decreased. The combination of all four techniques improved the ML model’s classification accuracy on the frog calls dataset by 94%. This study established a data augmentation approach to maximize the classification accuracy with sparse data of frog call recordings, thereby creating a possibility to build a real-world automated field frog species identifier system. Such a system can significantly help in the conservation of frog species in this vital biodiversity hotspot.
Read More...Predicting smoking status based on RNA sequencing data
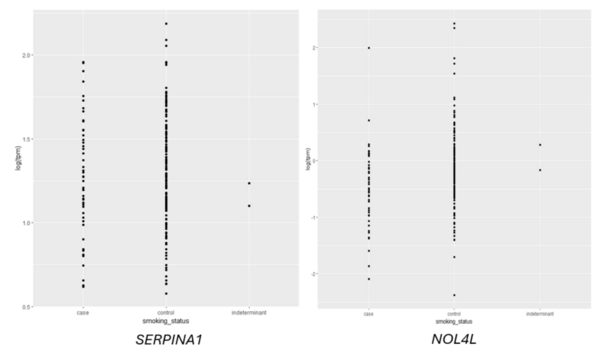
Given an association between nicotine addiction and gene expression, we hypothesized that expression of genes commonly associated with smoking status would have variable expression between smokers and non-smokers. To test whether gene expression varies between smokers and non-smokers, we analyzed two publicly-available datasets that profiled RNA gene expression from brain (nucleus accumbens) and lung tissue taken from patients identified as smokers or non-smokers. We discovered statistically significant differences in expression of dozens of genes between smokers and non-smokers. To test whether gene expression can be used to predict whether a patient is a smoker or non-smoker, we used gene expression as the training data for a logistic regression or random forest classification model. The random forest classifier trained on lung tissue data showed the most robust results, with area under curve (AUC) values consistently between 0.82 and 0.93. Both models trained on nucleus accumbens data had poorer performance, with AUC values consistently between 0.65 and 0.7 when using random forest. These results suggest gene expression can be used to predict smoking status using traditional machine learning models. Additionally, based on our random forest model, we proposed KCNJ3 and TXLNGY as two candidate markers of smoking status. These findings, coupled with other genes identified in this study, present promising avenues for advancing applications related to the genetic foundation of smoking-related characteristics.
Read More...Large Language Models are Good Translators
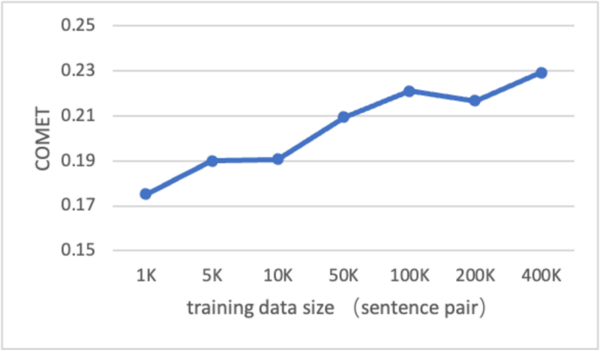
Machine translation remains a challenging area in artificial intelligence, with neural machine translation (NMT) making significant strides over the past decade but still facing hurdles, particularly in translation quality due to the reliance on expensive bilingual training data. This study explores whether large language models (LLMs), like GPT-4, can be effectively adapted for translation tasks and outperform traditional NMT systems.
Read More...Battling cultural bias within hate speech detection: An experimental correlation analysis
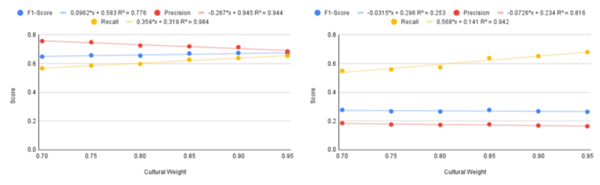
The authors develop a new method for training machine learning algorithms to differentiate between hate speech and cultural speech in online platforms.
Read More...Quantitative analysis and development of alopecia areata classification frameworks
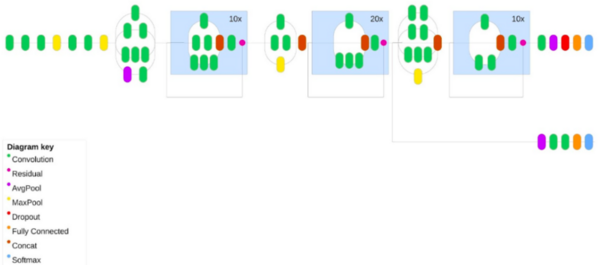
This article discusses Alopecia areata, an autoimmune disorder causing sudden hair loss due to the immune system mistakenly attacking hair follicles. The article introduces the use of deep learning (DL) techniques, particularly convolutional neural networks (CNN), for classifying images of healthy and alopecia-affected hair. The study presents a comparative analysis of newly optimized CNN models with existing ones, trained on datasets containing images of healthy and alopecia-affected hair. The Inception-Resnet-v2 model emerged as the most effective for classifying Alopecia Areata.
Read More...An optimal pacing approach for track distance events
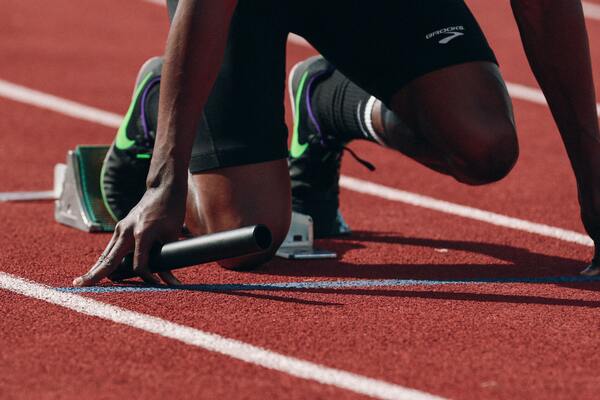
In this study, the authors use existing mathematical models to how high school athletes pace 800 m, 1600 m, and 3200 m distance track events compared to elite athletes.
Read More...Deep residual neural networks for increasing the resolution of CCTV images
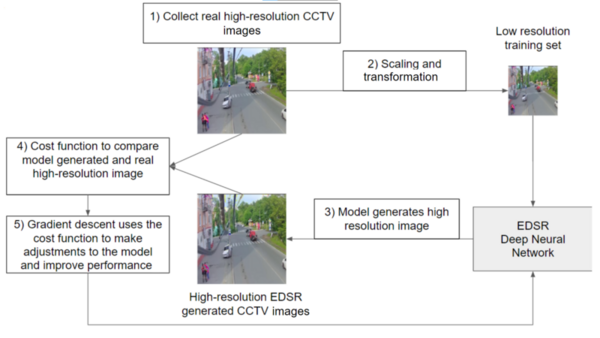
In this study, the authors hypothesized that closed-circuit television images could be stored with improved resolution by using enhanced deep residual (EDSR) networks.
Read More...The precision of machine learning models at classifying autism spectrum disorder in adults
.png)
Autism spectrum disorder (ASD) is hard to correctly diagnose due to the very subjective nature of diagnosing it: behavior analysis. Due to this issue, we sought to find a machine learning-based method that diagnoses ASD without behavior analysis or helps reduce misdiagnosis.
Read More...