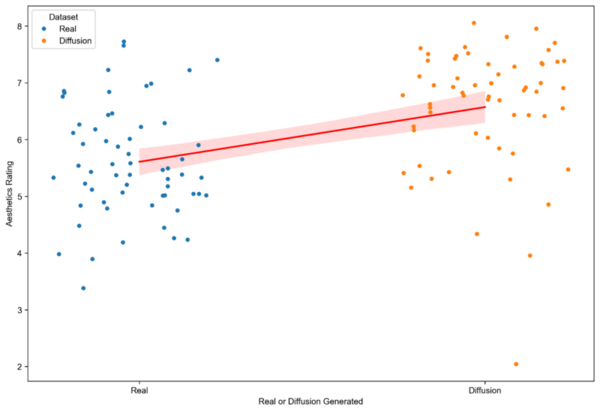
The authors develop a method for detecting fake AI-generated images from real images.
Read More...SpottingDiffusion: Using transfer learning to detect Latent Diffusion Model-synthesized images
A natural language processing approach to skill identification in the job market
The authors looked at using machine learning to identify skills needed to apply for certain jobs, specifically looking at different techniques to parse apart the text. They found that Bidirectional Encoder Representation of Transforms (BERT) performed best.
Read More...Uncovering the hidden trafficking trade with geographic data and natural language processing
The authors use machine learning to develop an evidence-based detection tool for identifying human trafficking.
Read More...Training neural networks on text data to model human emotional understanding
The authors train a neural network to detect text-based emotions including joy, sadness, anger, fear, love, and surprise.
Read More...Part of speech distributions for Grimm versus artificially generated fairy tales
Here, the authors wanted to explore mathematical paradoxes in which there are multiple contradictory interpretations or analyses for a problem. They used ChatGPT to generate a novel dataset of fairy tales. They found statistical differences between the artificially generated text and human produced text based on the distribution of parts of speech elements.
Read More...Using text embedding models as text classifiers with medical data
This article describes the classification of medical text data using vector databases and text embedding. Various large language models were used to generate this medical data for the classification task.
Read More...Rhythmic lyrics translation: Customizing a pre-trained language model using stacked fine-tuning
Neural machine translation (NMT) is a software that uses neural network techniques to translate text from one language to another. However, one of the most famous NMT models—Google Translate—failed to give an accurate English translation of a famous Korean nursery rhyme, "Airplane" (비행기). The authors fine-tuned a pre-trained model first with a dataset from the lyrics domain, and then with a smaller dataset containing the rhythmical properties, to teach the model to translate rhythmically accurate lyrics. This stacked fine-tuning method resulted in an NMT model that could maintain the rhythmical characteristics of lyrics during translation while single fine-tuned models failed to do so.
Read More...Open Source RNN designed for text generation is capable of composing music similar to Baroque composers
Recurrent neural networks (RNNs) are useful for text generation since they can generate outputs in the context of previous ones. Baroque music and language are similar, as every word or note exists in context with others, and they both follow strict rules. The authors hypothesized that if we represent music in a text format, an RNN designed to generate language could train on it and create music structurally similar to Bach’s. They found that the music generated by our RNN shared a similar structure with Bach’s music in the input dataset, while Bachbot’s outputs are significantly different from this experiment’s outputs and thus are less similar to Bach’s repertoire compared to our algorithm.
Read More...Comparing transformer and RNN models in BCIs for handwritten text decoding via neural signals
Brain-Computer Interface (BCI) allows users, especially those with paralysis, to control devices through brain activity. This study explored using a custom transformer model to decode neural signals into handwritten text for individuals with limited motor skills, comparing its performance to a traditional RNN-based BCI.
Read More...Depression detection in social media text: leveraging machine learning for effective screening
Depression affects millions globally, yet identifying symptoms remains challenging. This study explored detecting depression-related patterns in social media texts using natural language processing and machine learning algorithms, including decision trees and random forests. Our findings suggest that analyzing online text activity can serve as a viable method for screening mental disorders, potentially improving diagnosis accuracy by incorporating both physical and psychological indicators.
Read More...