Comparing transformer and RNN models in BCIs for handwritten text decoding via neural signals
(1) Horace Mann School, (2) Computer Science and Technology, University of Cambridge
https://doi.org/10.59720/24-027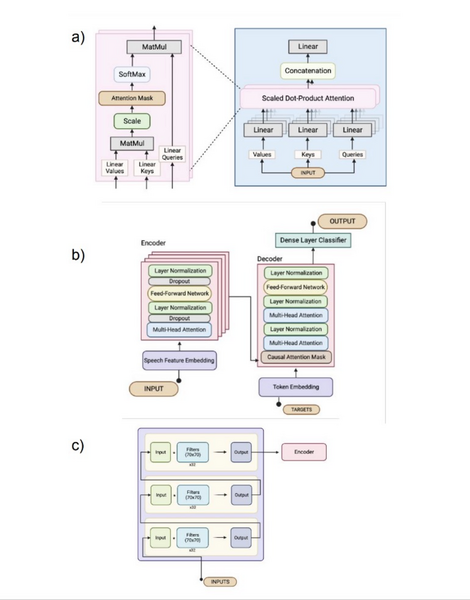
A Brain-Computer Interface (BCI) is a technology that enables the user to operate devices or manipulate objects solely through their brain activity, which is especially useful for paralyzed individuals in regaining communication. The purpose of this study is to explore the use of a custom transformer model in BCIs that translates the neural activity present when an individual with limited verbal and fine-motor skills attempts to handwrite. Previous studies have found that transformers performed better than recurrent neural networks (RNNs) in translation tasks similar to decoding neural signals into intended handwritten text. Due to this known benefit, we hypothesized that the transformer BCI would show promise through the recorded metrics. The neural signals of a tetraplegic individual attempting to handwrite were provided by existing research. We conducted four trials using training data with or without augmentation, as well as training the model to separately minimize training and validation loss. Compared with the original RNN BCI, the transformer model performed less favorably across all trials. Although the results do not indicate that the transformer currently outperforms an RNN BCI, it is important to note that further testing of the model’s capabilities is necessary before determining whether transformers can enhance BCI communication. Future testing could include training the model with a larger and more preferable dataset, training for a longer duration, comparing training times between the RNN and transformer, or assessing how the transformer improves with an offline autocorrect feature.
This article has been tagged with: