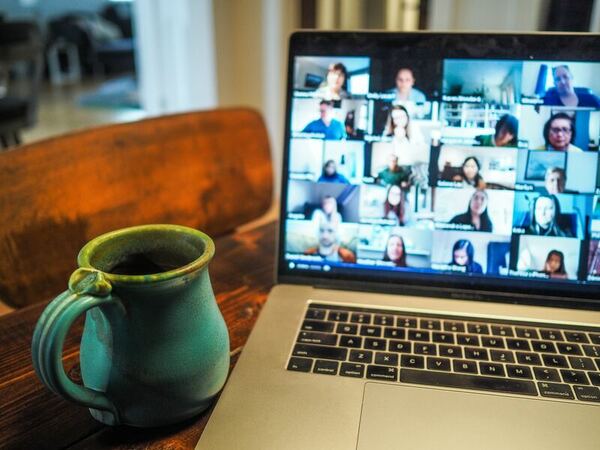
Here, seeking to understand how the COVID-19 pandemic affected the social interactions of junior high school students, the authors surveyed students, teachers, and parents. Contrary to their initial hypotheses, the authors found positive correlation between increased virtual contact during social isolation and in-person conflict and disregard for social norms after the pandemic. While the authors identified the limitations of their study, they suggest that further research into the effect of online interactions is becoming increasingly important.
Read More...