An improved video fingerprinting attack on users of the Tor network
(1) Padua Academy, Wilmington, Delaware, (2) Massachusetts Institute of Technology, Cambridge, Massachusetts
https://doi.org/10.59720/21-165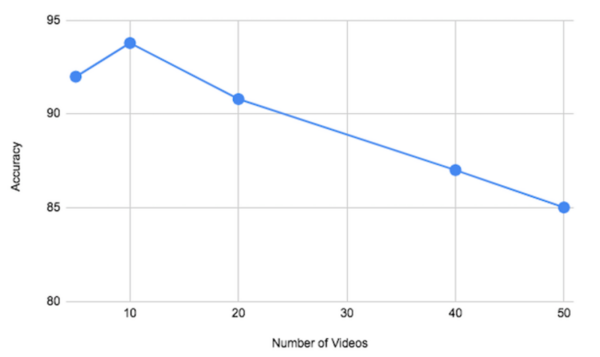
In today’s digital age, there is less and less doubt that companies, governments, and hackers track our online patterns and collect personal data. Thus, over 2 million users daily flock to the Tor network, which secures their online identities by encrypting their traffic. Although the Tor network is often considered secure, it is vulnerable to fingerprinting attacks that threaten users’ online privacy. Using machine learning, these attacks attempt to classify which web page the victim is visiting based on the page’s unique traffic patterns. Since video streaming makes up 80% of total downstream web traffic, we aim to explore how well this content can be fingerprinted in Tor. In this paper, we develop a new video fingerprinting model. Our model is based on a random forest classifier, a supervised machine learning algorithm that assembles decision trees for various samples and classifies based on their majority vote. Our model uses 247 features from video traces to exploit the burst patterns present in video traffic that are unique to each video. Our model is able to distinguish which one of the 50 videos a user is hypothetically watching on the Tor network with 85% accuracy, which outperforms the state-of-the-art, Deep Fingerprinting model’s accuracy of 55%. This demonstrates that video fingerprinting poses a serious threat to the privacy of Tor users. Our model performs better as it is adjusted to consider the bursts that are streamed from video traffic’s DASH protocol.
This article has been tagged with: