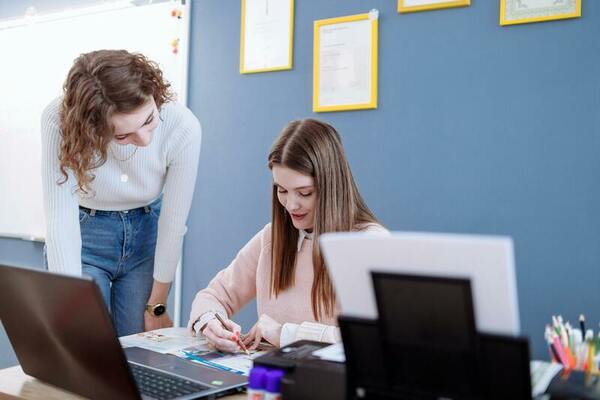
Middle school math forms the basis for advanced mathematical courses leading up to the university level. Large language models (LLMs) have the potential to power next-generation educational technologies, acting as digital tutors to students. The main objective of this study was to determine whether LLMs like ChatGPT, Bard, and Llama 2 can serve as reliable middle school math tutoring assistants on three tutoring tasks: hint generation, comprehensive solution, and exercise creation.
Read More...