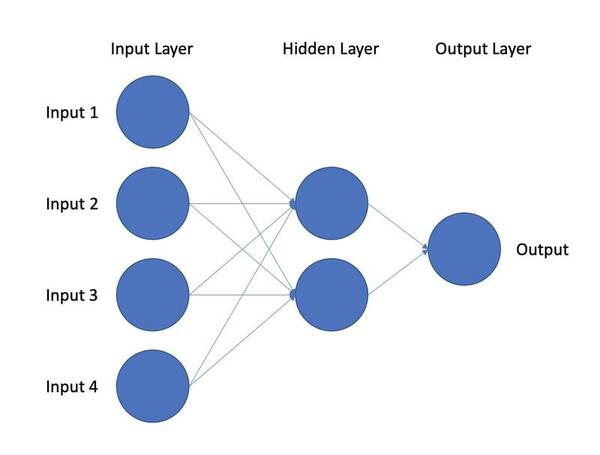
In this study, a deep learning model is used to classify post-traumatic stress disorder patients through novel markers to assist in finding candidate biomarkers for the disorder.
Read More...Post-Traumatic Stress Disorder (PTSD) biomarker identification using a deep learning model
In this study, a deep learning model is used to classify post-traumatic stress disorder patients through novel markers to assist in finding candidate biomarkers for the disorder.
Read More...A Crossover Study Comparing the Effect of a Processed vs. Unprocessed Diet on the Spatial Learning Ability of Zebrafish
The authors compared the short-term effects of processed versus unprocessed food on spatial learning and survival in zebrafish, given the large public concern regarding processed foods. By randomly assigning zebrafish to a diet of brine shrimp flakes (processed) or live brine shrimp (unprocessed), the authors show while there is no immediate effect on a fish's decision process between the two diets, there are significant correlations between improved learning and stress response with the unprocessed diet.
Read More...The influence of working memory on auditory category learning in the presence of visual stimuli
Here in an effort to better understand how our brains process and remember different categories of information, the authors assessed working memory capacity using an operation span task. They found that individuals with higher working memory capacity had higher overall higher task accuracy regardless of the type of category or the type of visual distractors they had to process. They suggest this may play a role in how some students may be less affected by distracting stimuli compared to others.
Read More...A machine learning approach for abstraction and reasoning problems without large amounts of data
While remarkable in its ability to mirror human cognition, machine learning and its associated algorithms often require extensive data to prove effective in completing tasks. However, data is not always plentiful, with unpredictable events occurring throughout our daily lives that require flexibility by artificial intelligence utilized in technology such as personal assistants and self-driving vehicles. Driven by the need for AI to complete tasks without extensive training, the researchers in this article use fluid intelligence assessments to develop an algorithm capable of generalization and abstraction. By forgoing prioritization on skill-based training, this article demonstrates the potential of focusing on a more generalized cognitive ability for artificial intelligence, proving more flexible and thus human-like in solving unique tasks than skill-focused algorithms.
Read More...Psychosocial impact of home-based learning among students during the COVID-19 Pandemic in Singapore
In this study, the authors surveyed a number of students in Singapore to determine how their experiences changed after the implementation of home-based learning during the COVID-19 pandemic.
Read More...EEG study of virtual learning demonstrates worsened learning outcomes and increased mirror neuron activation
In this article, Choi and Rossitto investigated the limitations of virtual learning by examining in-person dance learning compared to virtual dance learning while wearing EEG headsets. They found that in-person learners outperformed virtual learners and that virtual learners had higher mirror neuron activity as assessed by Mu rhythm power.
Read More...The influence of remote learning on sleep patterns of teenagers
In this study, the authors investigate the effect of remote learning (due to the COVID-19 pandemic) on sleeping habits amongst teenagers in Ohio. Using survey results, sleep habits and attitudes toward school were assessed before and after the COVID-19 pandemic.
Read More...Assessing and Improving Machine Learning Model Predictions of Polymer Glass Transition Temperatures
In this study, the authors test whether providing a larger dataset of glass transition temperatures (Tg) to train the machine-learning platform Polymer Genome would improve its accuracy. Polymer Genome is a machine learning based data-driven informatics platform for polymer property prediction and Tg is one property needed to design new polymers in silico. They found that training the model with their larger, curated dataset improved the algorithm's Tg, providing valuable improvements to this useful platform.
Read More...Artificial Intelligence Networks Towards Learning Without Forgetting
In their paper, Kreiman et al. examined what it takes for an artificial neural network to be able to perform well on a new task without forgetting its previous knowledge. By comparing methods that stop task forgetting, they found that longer training times and maintenance of the most important connections in a particular task while training on a new one helped the neural network maintain its performance on both tasks. The authors hope that this proof-of-principle research will someday contribute to artificial intelligence that better mimics natural human intelligence.
Read More...Optimizing data augmentation to improve machine learning accuracy on endemic frog calls
The mountain chain of the Western Ghats on the Indian peninsula, a UNESCO World Heritage site, is home to about 200 frog species, 89 of which are endemic. Distinctive to each frog species, their vocalizations can be used for species recognition. Manually surveying frogs at night during the rain in elephant and big cat forests is difficult, so being able to autonomously record ambient soundscapes and identify species is essential. An effective machine learning (ML) species classifier requires substantial training data from this area. The goal of this study was to assess data augmentation techniques on a dataset of frog vocalizations from this region, which has a minimal number of audio recordings per species. Consequently, enhancing an ML model’s performance with limited data is necessary. We analyzed the effects of four data augmentation techniques (Time Shifting, Noise Injection, Spectral Augmentation, and Test-Time Augmentation) individually and their combined effect on the frog vocalization data and the public environmental sounds dataset (ESC-50). The effect of combined data augmentation techniques improved the model's relative accuracy as the size of the dataset decreased. The combination of all four techniques improved the ML model’s classification accuracy on the frog calls dataset by 94%. This study established a data augmentation approach to maximize the classification accuracy with sparse data of frog call recordings, thereby creating a possibility to build a real-world automated field frog species identifier system. Such a system can significantly help in the conservation of frog species in this vital biodiversity hotspot.
Read More...