Post-Traumatic Stress Disorder (PTSD) biomarker identification using a deep learning model
(1) Thomas Jefferson High School for Science and Technology, Alexandria, Virginia, (2) University of Memphis, Memphis, Tennessee
https://doi.org/10.59720/21-161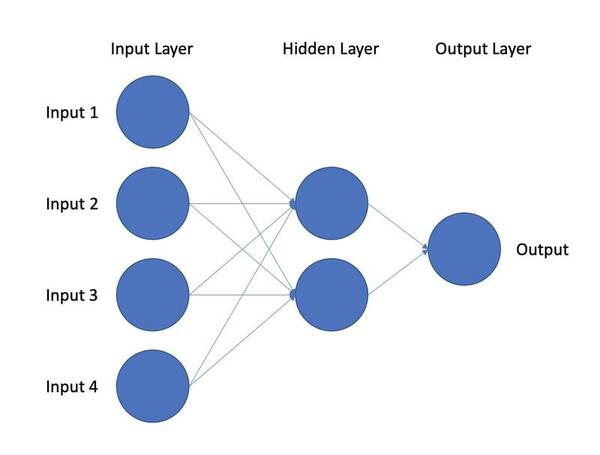
Post-traumatic stress disorder (PTSD) is a neuropsychological disorder in which individuals struggle to recover from a traumatizing event. It affects a significant population, including COVID-19 patients, frontline health workers, and war veterans. Given biases associated with self-assessment and diagnosis of PTSD, researchers are actively searching for unbiased biological markers (biomarkers) for predicting PTSD status. The Systems Biology of PTSD Consortium has collected candidate biomarkers for PTSD using molecular and clinical measurements of male war veterans between the ages of 20 and 60. PTSD-positive and negative subjects were separated based on the fourth edition of the Diagnostic and Statistical Manual of Mental Disorders (DSM-IV) Clinician Administered PTSD Scale (CAPS) scores, derived from structured interviews to measure an individual’s abundance of symptoms, such as re-experiencing, flashbacks, and hyperarousal. CAPS scores higher than 40 were considered PTSD-positive and below 20 were considered negative. We created artificial neural network models to classify PTSD-positive and negative individuals based on metabolomics, micro-RNA (miRNA), protein expression, endocrine markers, and DNA methylation datasets. Model training involved 64 iterations of a Bayesian Hyperparameter Optimization algorithm with 5-fold cross-validation. Each model was calibrated based on cross-validation performance and variance across iterations and then fit to the entire respective dataset (76 PTSD-positive, 76 PTSD-negative). We applied the trained models to an independent validation cohort to assess accuracy on unseen datasets. The top performing datasets from the validation cohort based on classification accuracy were metabolomics (65.2%) and protein expression (61.8%). We anticipate the candidate biomarkers identified in this and future studies will assist with the diagnosis of PTSD.
This article has been tagged with: