Assessing and Improving Machine Learning Model Predictions of Polymer Glass Transition Temperatures
(1) Joseph Wheeler High School, Marietta, Georgia, (2) School of Materials Science and Engineering, Georgia Institute of Technology, Atlanta, Georgia
https://doi.org/10.59720/19-097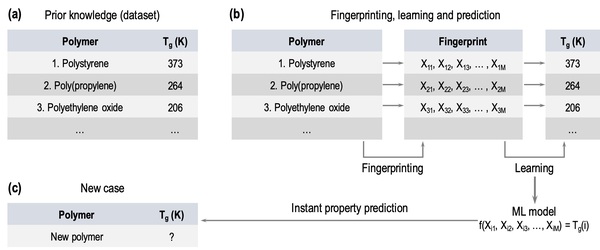
The success of the Materials Genome Initiative has led to opportunities for data-driven approaches for materials discovery. The recent development of Polymer Genome (PG), which is a machine learning (ML) based data-driven informatics platform for polymer property prediction, has significantly increased the efficiency of polymer design. Nevertheless, continuous expansion of the ‘training data’ is necessary to improve the robustness, versatility, and accuracy of the ML predictions. Accurate prediction of polymer properties, such as glass transition temperature (Tg), is advantageous for the design of polymers, particularly for high temperature applications. We hypothesized that by adding more data with increased chemical diversity to the dataset, the predictive capabilities of the PG model would improve. In order to test the performance and transferability of the predictive model for Tg (previously trained on a dataset of 450 polymers), we have carefully collected additional experimental Tg data for 871 polymers from multiple data sources. The Tg values predicted by the present PG models for the polymers in the newly collected dataset were compared directly with the experimental Tg to estimate the accuracy of the present model. Using the full dataset of 1321 polymers, a new ML model for Tg was built following past work. The root mean square error (RMSE) of prediction for the extended dataset, when compared to the earlier one, decreased to 27 K from 57 K, thereby supporting our initial hypothesis that increasing the dataset would improve the predictions. To further improve the performance of the Tg prediction model, we are continuing to accumulate new data and exploring new ML approaches.
This article has been tagged with: