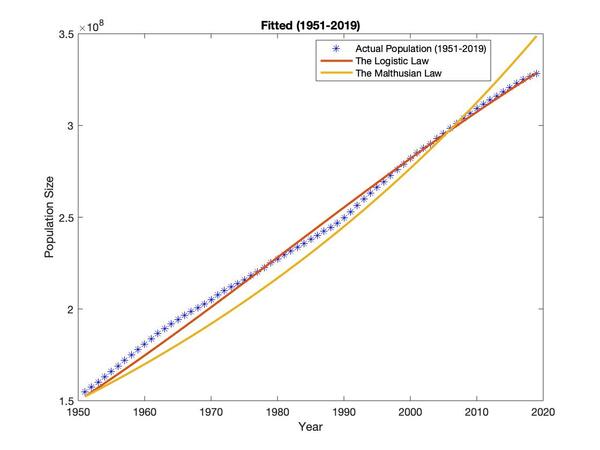
In this work, the authors investigate the accuracy with which two different population growth models can predict population growth over time. They apply the Malthusian law or Logistic law to US population from 1951 until 2019. To assess how closely the growth model fits actual population data, a least-squared curve fit was applied and revealed that the Logistic law of population growth resulted in smaller sum of squared residuals. These findings are important for ensuring optimal population growth models are implemented to data as population forecasting affects a country's economic and social structure.
Read More...