Genetic algorithm based features selection for predicting the unemployment rate of India
(1) St. Francis College, (2) ZAS Consultancy Services Ltd
https://doi.org/10.59720/22-252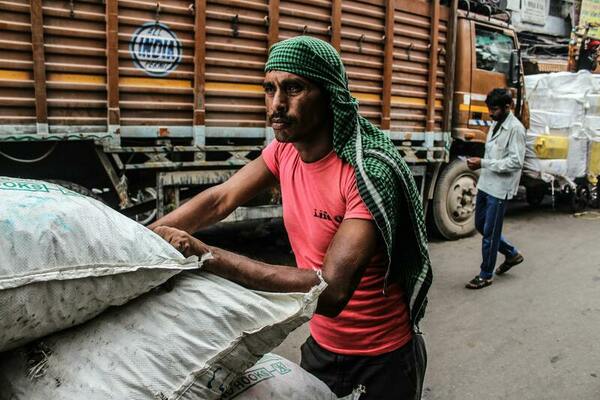
The forecasting of employment is critical for policymakers to prevent large impacts on modern life. Many studies have been conducted to predict the unemployment rate using time series models and structural models using data from advanced economy countries, but only a few studies have used data from developing countries. In addition, the two economic theories which focus on short-term inverse relationships between “unemployment and inflation” or “unemployment and gross domestic product” as described by the Phillips curve and Okun’s law, fail to predict the unemployment in low/middle-income countries and advanced economies undergoing stress. In this study, we have chosen India’s labor market, to test the hypothesis described by the Philips curve and Okun’s law because India’s economy exhibits the characteristics of low/middle-income countries with the majority of the labor force employed in agricultural and low-paying seasonal jobs. Given that the unemployment rate depends on a wide number of social and political factors we employed genetic algorithms (GA) for feature selection. Based on our limited literature survey, there were no research studies employing genetic algorithms to explain India’s labor market data so we have chosen India’s macroeconomic data to test the effectiveness of the GA model for features selection. Our results show that, for the chosen period, the Philips curve relationship doesn’t hold well for the Indian labor market. In addition, feature selection using the GA suggested that economic growth, household consumption, government spending and coal imports are the best macroeconomic variables to explain variation in the Indian labor market.
This article has been tagged with: