Forecasting air quality index: A statistical machine learning and deep learning approach
(1) Prospect High School, (2) AIClub
https://doi.org/10.59720/24-079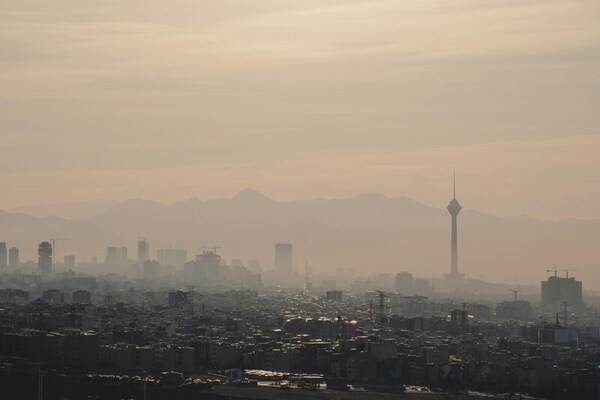
Air pollution is a serious issue that affects many people around the globe. It has many negative effects, especially on health, and is measured by the air quality index (AQI). India is one of the most polluted countries in the world, with over 660 million people living in areas with air pollution above the standard AQI. We hypothesize that traditional time series processing algorithms for forecasting, such as the Seasonal Autoregressive Integrated Moving Average (SARIMA), capture seasonal variations and can forecast future AQI levels better than the more common complex deep-learning models, like long short-term memory (LSTM) models. We used a dataset from the Central Pollution Control Board, the official portal of the Government of India that contains time series data for different cities. We created a forecasting model using the SARIMA and LSTM models. Our findings reveal that the SARIMA model effectively captures seasonal patterns in the data for all cities, except Chennai, predicting values with a minimal error margin. In contrast, the LSTM model, while comparable in some cases, generally exhibits poorer performance across more cities and underperforms compared to SARIMA even in its better scenarios. This trend is further evidenced by the root-mean-squared error (RMSE) results, where SARIMA consistently outperforms LSTM in all cities. Overall, our methodology demonstrates high accuracy, holding significant potential to positively impact numerous lives, and supports our hypothesis.
This article has been tagged with: