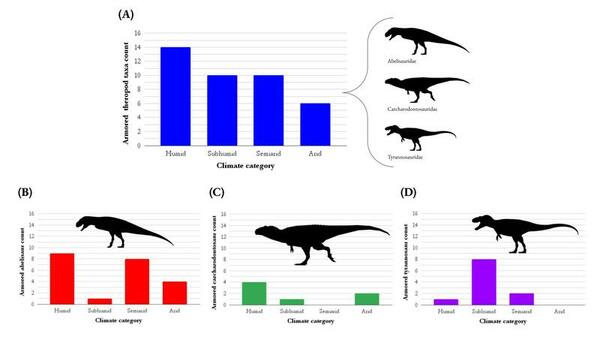
The facial integument, or external skin tissues, were assessed on set of dinosaurs from the Allosauroidea clade to test whether dermal patterns served specific functions.
Read More...Preliminary investigation of Allosauroidea facial integument and the evolution of theropod facial armor
The facial integument, or external skin tissues, were assessed on set of dinosaurs from the Allosauroidea clade to test whether dermal patterns served specific functions.
Read More...Are Age and Sex Related to Emotion Recognition Ability in Children and Teenagers?
Humans have a natural ability to recognize emotional cues from the facial expressions of others, as a crucial evolutionary trait to navigate social interactions. This ability likely develops through normal development and social experience, but it is unclear how much influence age and sex have in emotional facial recognition (EFR). In this study, the authors investigate EFR in children and teenagers, and look at whether accurate emotional recognition does occur more in males or females.
Read More...Propagation of representation bias in machine learning
Using facial recognition as a use-case scenario, we attempt to identify sources of bias in a model developed using transfer learning. To achieve this task, we developed a model based on a pre-trained facial recognition model, and scrutinized the accuracy of the model’s image classification against factors such as age, gender, and race to observe whether or not the model performed better on some demographic groups than others. By identifying the bias and finding potential sources of bias, his work contributes a unique technical perspective from the view of a small scale developer to emerging discussions of accountability and transparency in AI.
Read More...Physical Appearance and Its Effect on Trust
Do different physical traits affect teenagers’ initial trust of an unknown person? Would they give greater trust to women and people of similar ethnicity? To test these hypotheses, the authors developed a survey to determine the sets of physical characteristics that affect a person's trustworthiness. They found that gender and expression were the main physical traits associated with how trustworthy an individual looks, while ethnicity was also important.
Read More...Uncovering mirror neurons’ molecular identity by single cell transcriptomics and microarray analysis
In this study, the authors use bioinformatic approaches to characterize the mirror neurons, which are active when performing and seeing certain actions. They also investigated whether mirror neuron impairment was connected to neural degenerative diseases and psychiatric disorders.
Read More...Racial disparities in school discipline in Collier County, Florida
Here, the authorized analyzed data from the Florida Department of Education Office of Safe Schools regarding disciplinary outcomes in Collier County public schools. They reported that Black Students were more likely to receive both in-school and out-of-school suspensions than White students, which they concluded suggests racial inequities in school discipline that requires addressing as a society.
Read More...Racial and gender disparities in the portrayal of lawyers and physicians on television
Powered by the sociological framework that exposure to television bleeds into social biases, limiting media representation of women and minority groups may lead to real-world implications and manifestations of racial and gender disparities. To address this phenomenon, the researchers in this article take a look at primetime fictional representation of minorities and women as lawyers and physicians and compare television representation to census data of the same groups within real-world legal and medical occupations. The authors maintain the hypothesis that representation of female and minority groups as television lawyers and doctors is lower than that of their white male counterparts relative to population demographics - a trend that they expect to also be reflected in actual practice. With fictional racial and gender inequalities and corresponding real-world trends highlighted within this article, the researchers call for address towards representation biases that reinforce each other in both fictional and non-fictional spheres.
Read More...Sri Lankan Americans’ views on U.S. racial issues are influenced by pre-migrant ethnic prejudice and identity
In this study, the authors examined how Sri Lankan Americans (SLAs) view racial issues in the U.S. The main hypothesis is that SLAs, as a minority in the U.S., are supportive of the Black Lives Matter movement and its political goal, challenging the common notion that SLAs are anti-Black. The study found that a majority of SLAs believe the U.S. has systemic racism, favor BLM, and favor affirmative action. IT also found that Tamil SLAs have more favorable views of BLM and affirmative action than Sinhalese SLAs.
Read More...Don’t Waste the Medical Waste: Reducing Improperly Classified Hazardous Waste in a Medical Facility
Hemani et al. tackled the problem of rampant hospital waste by implementing staff training to help inform hospital workers about proper waste disposal. The authors observed a significant increase in proper waste disposal after the training, showing that simple strategies, such as in-person classroom training and posters, can have a profound effect on limiting improper waste handling.
Read More...The Prevalence of White Guilt Among American High School Students
Racial inequality has been a major issue throughout the history of the United States. In recent years, however, especially with the election of America's first black president, many have claimed that we have made progress and are moving towards a post-racial society. The authors of this study sought to test that claim by evaluating whether high school age students still experience a phenomenon known as white guilt. White guilt is defined as remorse or shame felt by people of Caucasian descent about racial inequality.
Read More...Search articles by title, author name, or tags