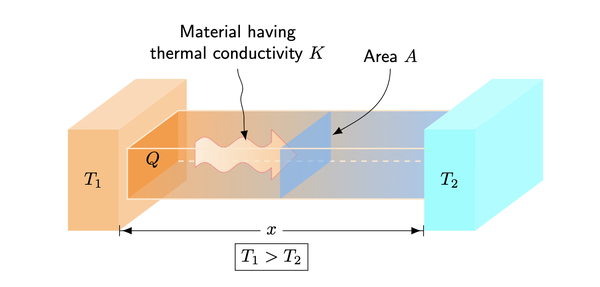
In this experiment, the authors modify the heat equation to account for imperfect insulation during heat transfer and compare it to experimental data to determine which is more accurate.
Read More...Heat conduction: Mathematical modeling and experimental data
In this experiment, the authors modify the heat equation to account for imperfect insulation during heat transfer and compare it to experimental data to determine which is more accurate.
Read More...Estimating the liquid jet breakdown height using dimensional analysis with experimental evidence
These authors mathematically deduce a model that explains the interesting (and unintuitive) physical phenomenon that occurs when water falls.
Read More...Open Source RNN designed for text generation is capable of composing music similar to Baroque composers
Recurrent neural networks (RNNs) are useful for text generation since they can generate outputs in the context of previous ones. Baroque music and language are similar, as every word or note exists in context with others, and they both follow strict rules. The authors hypothesized that if we represent music in a text format, an RNN designed to generate language could train on it and create music structurally similar to Bach’s. They found that the music generated by our RNN shared a similar structure with Bach’s music in the input dataset, while Bachbot’s outputs are significantly different from this experiment’s outputs and thus are less similar to Bach’s repertoire compared to our algorithm.
Read More...Design and in silico screening of analogs of rilpivirine as novel non-nucleoside reverse transcriptase inhibitors (NNRTIs) for antiretroviral therapy
In this study, the authors use high-throughput virtual screening to design and evaluate a set of non-nucleoside reverse transcriptase inhibitors for binding affinity to the protein reverse transcriptase. These studies have important applications toward HIV therapies.
Read More...Design and implementation of a cryptographically secure electronic voting infrastructure
In this study, the authors present proposed cryptographic controls for election sites with the hypothesis that this will mitigate risk and remediate vulnerabilities.
Read More...Reactivity-informed design, synthesis, and Michael addition kinetics of C-ring andrographolide analogs
Here, based on the identification of androgapholide as a potential therapeutic treatment against cancer, Alzheimer's disease, diabetes, and multiple sclerosis, due to its ability to inhibit a signaling pathway in immune system function, the authors sought ways to optimize the natural product human systems by manipulating its chemical structure. Through the semisynthesis of a natural product along with computational studies, the authors developed an understanding of the kinetic mechanisms of andrographolide and semisynthetic analogs in the context of Michael additions.
Read More...Battling cultural bias within hate speech detection: An experimental correlation analysis
The authors develop a new method for training machine learning algorithms to differentiate between hate speech and cultural speech in online platforms.
Read More...The design of Benzimidazole derivatives to bind to GDP-bound K-RAS for targeted cancer therapy
In this study, the authors looked at a proto-oncogene, KRAS, and searched for molecules that are predicted to be able to bind to the inactive form of KRAS. They found that a modified version of Irbesartan, a derivative of benzimidazole, showed the best binding to inactive KRAS.
Read More...Factors Influencing Muon Flux and Lifetime: An Experimental Analysis Using Cosmic Ray Detectors
Muons, one of the fundamental elementary particles, originate from the collision of cosmic rays with atmospheric particles and are also generated in particle accelerator collisions. In this study, Samson et al analyze the factors that influence muon flux and lifetime using Cosmic Ray Muon Detectors (CRMDs). Overall, the study suggests that water can be used to decrease muon flux and that scintillator orientation is a potential determinant of the volume of data collected in muon decay studies.
Read More...Modular mimics of neuroactive alkaloids - design, synthesis, and cholinesterase inhibitory activity of rivastigmine analogs
Naturally occurring neuroactive alkaloids are often studied for their potential to treat Neurological diseases. This team of students study Rivastigmine, a potent cholinesterase inhibitor that is a synthetic analog of physostigmine, which comes from the Calabar bean plant Physostigma venenosum. By comparing the effects of optimized synthetic analogs to the naturally occurring alkaloid, they determine the most favorable analog for inhibition of acetylcholinesterase (AChE), the enzyme that breaks down the neurotransmitter acetylcholine (ACh) to terminate neuronal transmission and signaling between synapses.
Read More...Search articles by title, author name, or tags