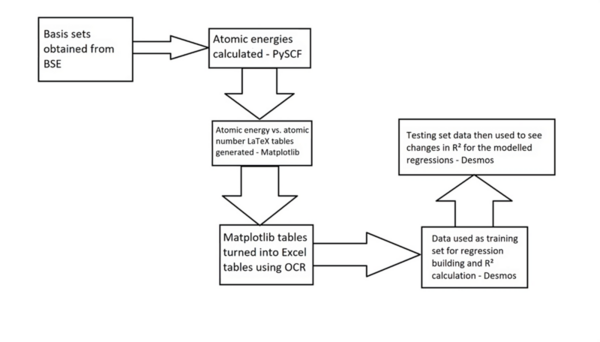
The energy of an atom is extremely useful in nuclear physics and reaction mechanism pathway determination but is challenging to compute. This work aimed to synthesize regression models for Pople Gaussian expansions of Slater-type Orbitals (STO-nG) atomic energy vs. atomic number scatter plots to allow for easy approximation of atomic energies without using computational chemistry methods. The data indicated that of the regressions, sinusoidal regressions most aptly modeled the scatter plots.
Read More...