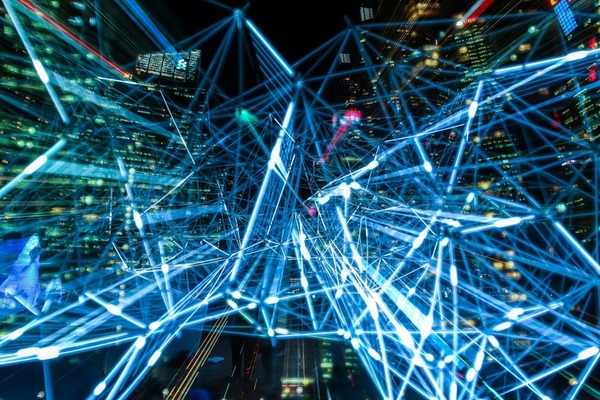
Reinforcement learning (RL) is a form of machine learning that can be harnessed to develop artificial intelligence by exposing the intelligence to multiple generations of data. The study demonstrates how reply buffer reward mechanics can inform the creation of new pruning methods to improve RL efficiency.
Read More...