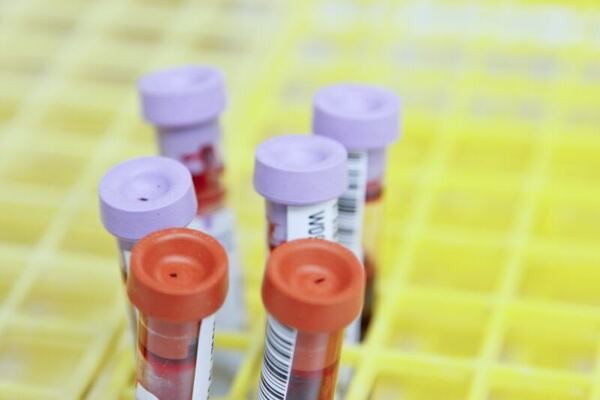
Here, recognizing the difficulty associated with tracking the progression of dementia, the authors used machine learning models to predict between the presence of cognitive normalcy, mild cognitive impairment, and Alzheimer's Disease, based on blood DNA methylation levels, sex, and age. With four machine learning models and two dataset dimensionality reduction methods they achieved an accuracy of 53.33%.
Read More...