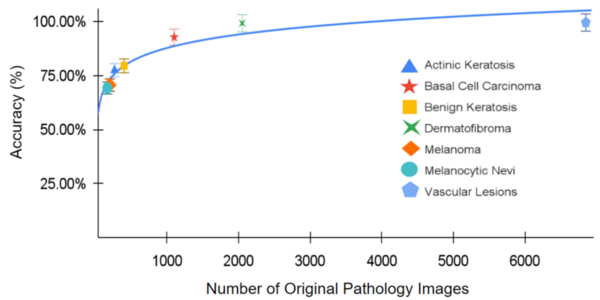
In this study, the authors developed and assessed the accuracy of a machine learning algorithm to identify skin cancers using images of biopsies.
Read More...A novel CNN-based machine learning approach to identify skin cancers
In this study, the authors developed and assessed the accuracy of a machine learning algorithm to identify skin cancers using images of biopsies.
Read More...Studying habitability of the exoplanents Kepler-504 b, Kepler-315 b, and Kepler-315 c
The authors explore how similar exoplanets are to Earth and whether they could be inhabited by humans and other living organisms.
Read More...A novel deep learning model for visibility correction of environmental factors in autonomous vehicles
Intelligent vehicles utilize a combination of video-enabled object detection and radar data to traverse safely through surrounding environments. However, since the most momentary missteps in these systems can cause devastating collisions, the margin of error in the software for these systems is small. In this paper, we hypothesized that a novel object detection system that improves detection accuracy and speed of detection during adverse weather conditions would outperform industry alternatives in an average comparison.
Read More...Post-Traumatic Stress Disorder (PTSD) biomarker identification using a deep learning model
In this study, a deep learning model is used to classify post-traumatic stress disorder patients through novel markers to assist in finding candidate biomarkers for the disorder.
Read More...Assessing grass water use efficiency through smartphone imaging and ImageJ analysis
Overwatering and underwatering grass are widespread issues with environmental and financial consequences. This study developed an accessible method to assess grass water use efficiency (WUE) combining smartphone imaging with open access color unmixing analysis. The method can be applied in automated irrigation systems or apps, providing grass WUE assessment for regular consumer use.
Read More...A novel approach for early detection of Alzheimer’s disease using deep neural networks with magnetic resonance imaging
In the battle against Alzheimer's disease, early detection is critical to mitigating symptoms in patients. Here, the authors use a collection of MRI scans, layering with deep learning computer modeling, to investigate early stages of AD which can be hard to catch by human eye. Their model is successful, able to outperform previous models, and detected regions of interest in the brain for further consideration.
Read More...Comparison of the ease of use and accuracy of two machine learning algorithms – forestry case study
Machine learning algorithms are becoming increasingly popular for data crunching across a vast area of scientific disciplines. Here, the authors compare two machine learning algorithms with respect to accuracy and user-friendliness and find that random forest algorithms outperform logistic regression when applied to the same dataset.
Read More...Developing a Method to Remove Inorganic Arsenic from Rice with Natural Substances
In this study, the authors tested different approaches for removing arsenic from rice. Due to higher arsenic levels in water, some areas grow rice with higher levels as well. This is a health hazard and so developing methods to remove arsenic from the rice will be helpful to many. Using a rapid arsenic kit, the authors found that activated charcoal was the most effective at removing arsenic from rice.
Read More...An analysis of the distribution of microplastics along the South Shore of Long Island, NY
This study is focused on the distribution of microplastics in Long Island, NY. Microplastics are plastic particles that measure less than 5 mm in length and pose an environmental risk due to their size, composition, and ubiquitous location in the marine environment. Focusing on the South Shore of Long Island, the authors investigated the locations and concentrations of microplastics at four locations along the shore line. While they did not find significant differences in the number of microplastics per location, there were microplastics at all four locations. This finding is important to drive future research and environmental policy as well.
Read More...Geographic Distribution of Scripps National Spelling Bee Spellers Resembles Geographic Distribution of Child Population in US States upon Implementation of the RSVBee “Wildcard” Program
The Scripps National Spelling Bee (SNSB) is an iconic academic competition for United States (US) schoolchildren, held annually since 1925. However, the sizes and geographic distributions of sponsored regions are uneven. One state may send more than twice as many spellers as another state, despite similar numbers in child population. In 2018, the SNSB introduced a wildcard program known as RSVBee, which allowed students to apply to compete as a national finalist, even if they did not win their regional spelling bee. In this study, the authors tested the hypothesis that the geographic distribution of SNSB national finalists more closely matched the child population of the US after RSVBee was implemented.
Read More...