Machine Learning Algorithm Using Logistic Regression and an Artificial Neural Network (ANN) for Early Stage Detection of Parkinson’s Disease
(1) duPont Manual High School, (2) W University of Louisville
https://doi.org/10.59720/20-015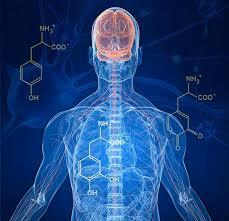
Parkinson’s disease (PD) is the second most common neurodegenerative disease. Despite the prevalence of PD, diagnosing PD is expensive, requires specialized testing, and is often inaccurate. Moreover, diagnosis is often made late in the disease course when treatments are less effective. Since one of the earliest symptoms of PD are changes in voice patterns, we employed machine learning algorithms to detect these abnormalities. Using existing voice data from patients with PD and healthy controls, we created and trained two different algorithms: one using logistic regression and another employing an artificial neural network (ANN). The inputs for these algorithms were two statistical measures of voice patterns: Pitch Period Entropy (PPE) and Spread1. Both algorithms were successfully able to discriminate between PD patients and healthy controls with F2 scores > 0.93. Moreover, we found that the time from diagnosis had no impact on the performance of our models. Thus, we report the creation of two models that can reliably and accurately identify the voice patterns characteristic of PD. Our findings suggest that it is possible to diagnose PD by analyzing voice patterns, which would enable disease screening that is cheap, accessible, and accurate.
This article has been tagged with: