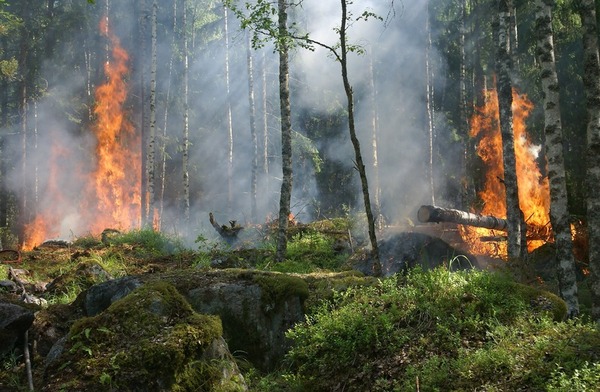
This study hypothesized that a machine learning model could accurately predict the severity of California wildfires and determine the most influential meteorological factors. It utilized a custom dataset with information from the World Weather Online API and a Kaggle dataset of wildfires in California from 2013-2020. The developed algorithms classified fires into seven categories with promising accuracy (around 55 percent). They found that higher temperatures, lower humidity, lower dew point, higher wind gusts, and higher wind speeds are the most significant contributors to the spread of a wildfire. This tool could vastly improve the efficiency and preparedness of firefighters as they deal with wildfires.
Read More...