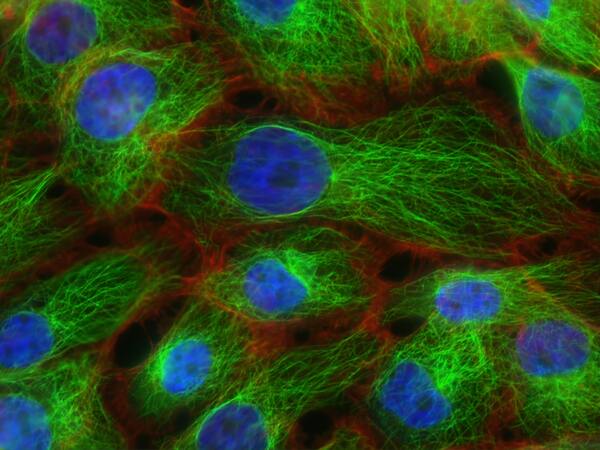
Machine learning and deep learning techniques can be used to predict the early onset of breast cancer. The main objective of this analysis was to determine whether machine learning algorithms can be used to predict the onset of breast cancer with more than 90% accuracy. Based on research with supervised machine learning algorithms, Gaussian Naïve Bayes, K Nearest Algorithm, Random Forest, and Logistic Regression were considered because they offer a wide variety of classification methods and also provide high accuracy and performance. We hypothesized that all these algorithms would provide accurate results, and Random Forest and Logistic Regression would provide better accuracy and performance than Naïve Bayes and K Nearest Neighbor.
Read More...