Minimizing distortion with additive manufacturing parts using Machine Learning
(1) Valley Christian High School, (2) Stanford University
https://doi.org/10.59720/24-086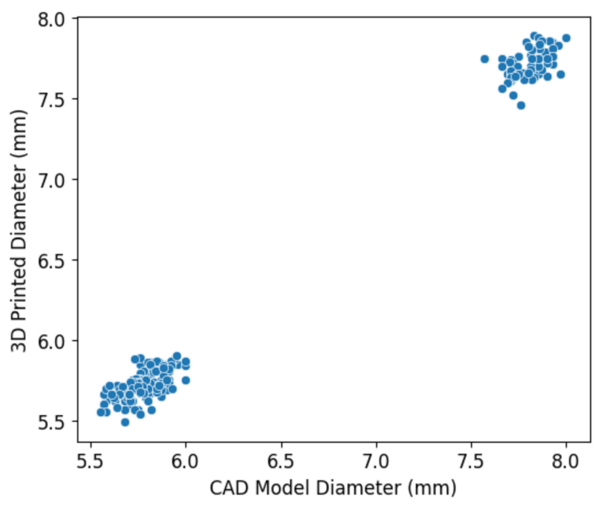
Additive manufacturing technologies are increasingly being used in both academic and corporate settings as an efficient and precise method of manufacturing. However, the accuracy of affordable 3D printing methods is compromised by distortion of the plastic filament. These inaccurate 3D prints may not be usable; incorrect sizing of specific features, such as holes, can mean the part does not fit in the larger assembly and would need to be discarded. This can contribute to plastic pollution due to mismanagement, compounded by difficult access to the recycling process of the most popular filament, Polylactic Acid (PLA). In this study, we investigated to what extent the distortion introduced in the additive manufacturing process can be predicted and minimized. We hypothesized that predicting the computer-aided design (CAD) dimensions based on historical print misalignments would be effective in minimizing this distortion. We used a histogram-based gradient boosting regressor and trained it on 3D printing data of hole dimensions printed in PLA filament, obtained from Mendeley Data. Our model predicts the CAD geometry that results in the desired final print dimension, accounting for distortion. The model had a root mean squared error of 0.0614 mm, a mean absolute error of 0.0452 mm, and an R2 score of 99.617%. These results can be used to minimize inaccuracies and prevent waste production.
This article has been tagged with: